Information Technology Reference
In-Depth Information
ONTECTAS
ONTECTAS
BB
BB
Schmitz
Schmitz
LFZ
LFZ
DAG
DAG
0.9
0.9
ONTECTAS
ONTECTAS
BB
BB
Schmitz
Schmitz
LFZ
LFZ
DAG
DAG
0.45
0.45
0.8
0.8
0.4
0.4
0.7
0.7
0.35
0.35
0.6
0.6
0.3
0.3
0.5
0.5
0.25
0.25
0.4
0.4
0.2
0.2
03
0.3
0.15
0.2
0.1
0.1
0.05
0
0
Del.icio.us
CiteULike
LibraryThing
Del.icio.us
CiteULike
LibraryThing
(b) Relative Recall —
is-a
(a) Precision
ONTECTAS
ONTECTAS
BB
BB
Schmitz
Schmitz
LFZ
LFZ
DAG
DAG
ONTECTAS
ONTECTAS
BB
BB
Schmitz
Schmitz
LFZ
LFZ
DAG
DAG
2.5
2.5
7
7
6
6
2
2
5
5
1.5
1.5
4
4
3
3
1
1
2
0.5
1
0
0
Del.icio.us
CiteULike
LibraryThing
Del.icio.us
CiteULike
LibraryThing
(c) Average Depth
(d) Maximum Depth
Fig. 1.
Comparison of ONTECTAS to other algorithms for different metrics. Lower
bars show
is-a
relationships and higher bars show “any” relationships.
Figure 1(a) shows the algorithms' precision for both
is-a
relationships (the
lower bars) and
relationships, the
precision of ONTECTAS is 0.50 for Del.icio.us, 0.48 for LibraryThing and 0.29
for CiteULike. ONTECTAS outperforms the precision of all other algorithms on
all datasets. We also compare our precision on
any
relationships (the higher bars); for
is-a
is-a
with that on
is-a
+
any
for
the other algoritms since they do not distinguish
.Eventhen
ONTECTAS outperforms the other algorithms in del.icio.us and CiteULike. On
LibraryThing, the performance is close to the winner.
Figure 1(b) compares the algorithms' relative recall for
is-a
from non-
is-a
relationships.
ONTECTAS is the best performer for all three datasets. One reason for DAG-
ALG's bad relative recall is that it detected many popular tags such as “web”
and “software” as subjective tags, and pruned them before discovering the edges.
BB had relatively low precision and recall in CiteULike because it detected
many relationships with the tag “no-tag”, which is a popular tag rather than
an ontological tag. ONTECTAS performs the best for relative recall for
is-a
any
relationships [20].
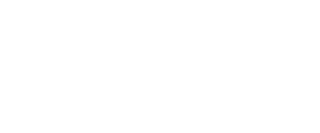
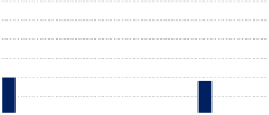

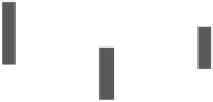
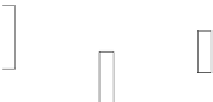


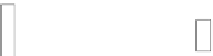



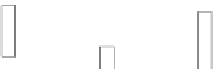
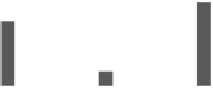


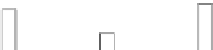








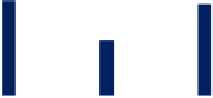
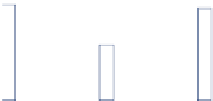

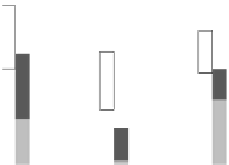
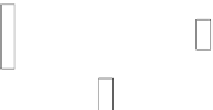
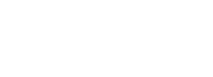

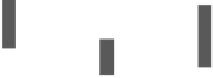

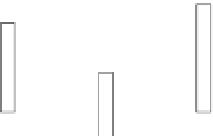


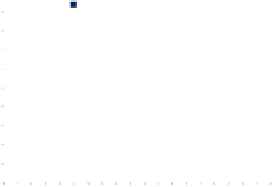





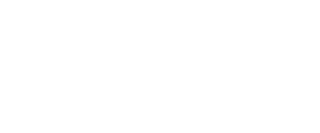

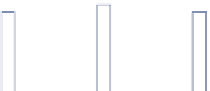

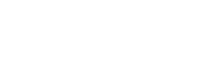
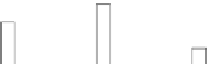









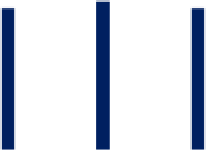
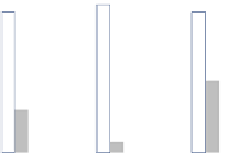
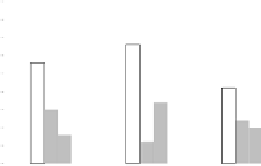







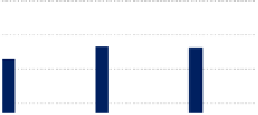
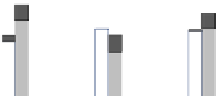
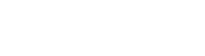
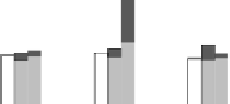



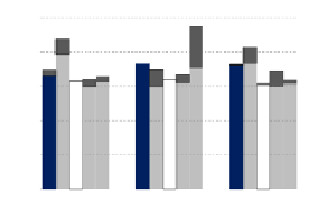



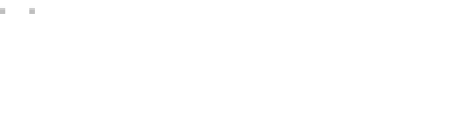
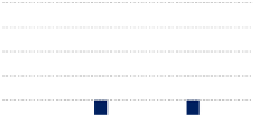





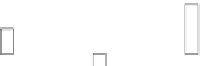





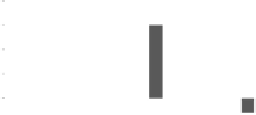


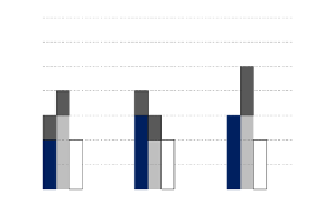
















