Geography Reference
In-Depth Information
Table 7.1
River parameters controlling green laser waveforms.
River Property
Waveform Impact
Impact on LiDAR Measurement Accuracy
Water velocity
Decrease backscatter signal power
weak
•
•
Increase or decrease the surface
backscatter signal power
increase water-surface altimetry accuracy
Water surface roughness
•
•
decrease very shallow water
depth-measurement capacities
•
(with an 'optimum')
•
Water surface slope
decrease surface backscatter signal power
enlarge LiDAR measure resolution
•
•
decrease bottom backscatter signal power
decrease very shallow water
depth-measurement capacities
•
•
Water turbidity (from sediment,
dissolve organic matter or
phytoplankton)
decrease bottom backscatter signal power
decrease deep water depth-measurement
capacities
•
•
increase water column backscatter signal
power
•
decrease bathymetry accuracy
•
Increase bottom backscatter signal power
with reflective materials
increase bathymetry accuracy in case of
reflective materials
River bottom material
•
•
River bottom roughness
decrease bottom backscatter signal power
decrease very shallow water
depth-measurement capacities
•
•
River bottom slope
decrease bottom backscatter signal power
decrease very shallow water
depth-measurement capacities
•
•
Presence of algae
Increase water column backscatter signal
power
decrease bathymetry accuracy
•
•
undercutting region which compromises depth accuracy.
Measurement biases can be magnified by physical and
biological properties inherent to riverine environments.
Some of the main physical and biological properties of
rivers controlling the collected signal power over time,
i.e., waveform
P
(
t
), are listed in Table 7.1.
The waveforms are the raw data of bathymetric LiDAR,
from which derivative variables can be estimated (see
Section 7.4). For instance, information such as water
depth is derived from the surface peak (t
1
) and bottom
(t
2
) positioning in a waveform through (in the case of a
nadir laser beam):
assist in evaluating the performance and accuracy of
various signal processing algorithms to retrieve water
parameters. For example, Zege et al. (2004) developed the
software AOLS (Airborne Oceanic Lidar Simulator) and
verified retrieval techniques using analytical inversion
of the Raman lidar equation from a set of Raman
waveform simulations. Another example can be found
in Lesaignoux et al. (2007): the use of a huge set
of simulated green waveforms as examples shown in
Figure 7.2b, allowed the assessment of the performance
and limitations for very shallow-water bathymetry using
a bi-Gaussian fitting process on waveforms.
c
2
(
t
2
−
t
1
)
2
where
c
2
is light speed in water
(2
.
25 10
8
m.s
−
1
)
.
d
=
7.2.2.2 Multispectral systems
As shown in section 7.2.2.1, a unique characteristic of
bathymetric LiDARs is the use of different wavelengths
in transmission or reception. These different wavelengths
are usually 1,064 and 532 nm in transmission, and in the
SHOALS (Scanning Hydrographic Operational Airborne
LiDAR Survey) LiDAR system (Irish and Lillycrop, 1999),
the Raman wavelength of 634 nm is also analysed in the
To perform simulations of bathymetric waveforms
and derivative information, simulation based on these
equations have been implemented (Zege et al., 2004;
Lesaignoux et al., 2007). These tools permit the explo-
ration of LiDAR signals in various aquatic conditions
and
system
design
configurations.
These
tools
also
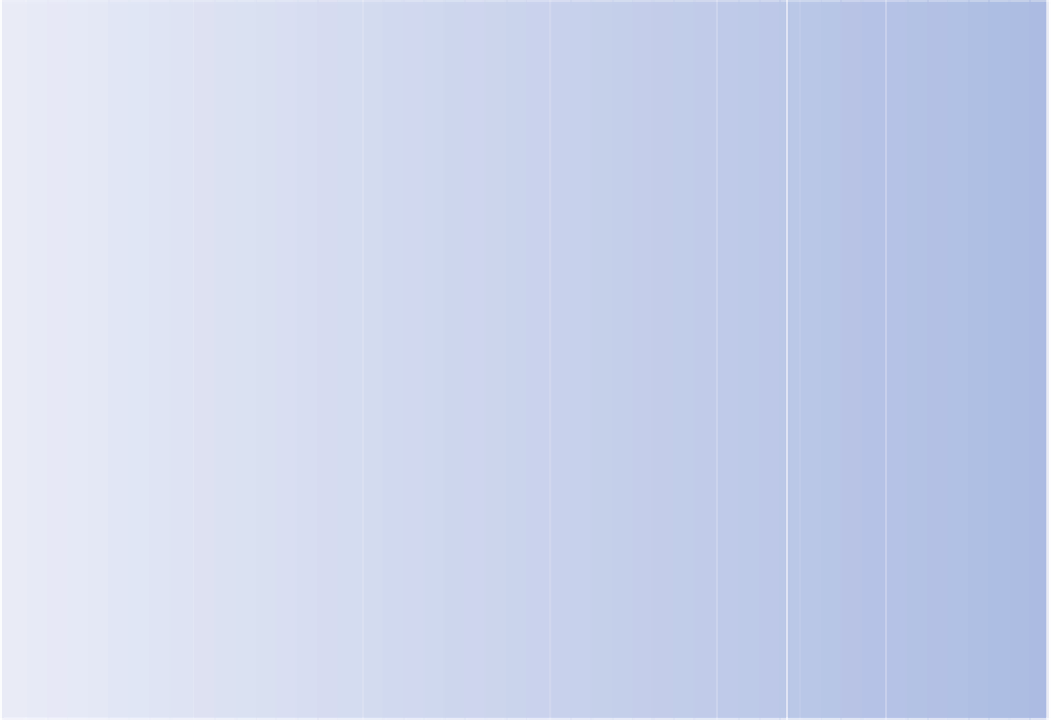



Search WWH ::

Custom Search