Geoscience Reference
In-Depth Information
Another important parameter is the Prandtl number,
defined by
interaction between mode
m
=2and
m
= 3 occurs. Fur-
thermore, structural (or shape) vacillations are found at
high Taylor numbers (Figure 17.3, lower row), where cold
cells separate from the inner wall, move radially outward,
and then return to the inner cylinder while their outer
boundary largely remains unaffected.
While the complex flow patterns in the first transition
zone might be identified as a superposition of two coex-
isting waves with different zonal wave numbers and phase
speeds, denoted as
interference vacillation
(IV), which was
found to occur in experiments with a free surface [
Pfeffer
and
Fowlis
, 1968;
Kaiser
, 1970;
Harlander et al.
, 2011] as
in rigid-lid experiments [
Früh and Read
, 1997], the struc-
tural vacillations observed at higher Taylor numbers could
be consistent with an oscillation of a higher radial mode
of the same azimuthal wave as observed in experiments by
Pfeffer et al.
[1980] and described theoretically by
Weng
et al.
[1986].
Pr =
ν
κ
,
(17.4)
where
κ
is thermal conductivity and Pr describes the phys-
ical properties of the fluid, with Pr = 7.16 as the appro-
priate value for water at 20
◦
C (see chapter 2 this volume
and
Fowlis and Hide
[1965] for more details on the effect
of the Prandtl number).
17.2.3. Flow regimes
By keeping
T
= 7.5 K constant and varying
in
the range 3
10
8
, the regime diagram
typically shows specific flow regimes characterized by the
azimuthal wave number 0
10
6
×
<
Ta
<
3
×
≤
m
≤
4, i.e., the axisymmet-
10
6
10
6
,the
ric
m
= 0 basic flow at 3
×
<
Ta
<
6
×
10
6
≤
≤
×
wave flow regime with 2
m
3 at about 6
<
10
8
, and the regime with structural vacillations
(called irregular wave regime when the wave structure is
deformed in an irregular way) with dominant wave
m
=4
at higher Taylor numbers (see Figure 17.2). Note, that
m
max
= 4 is in good agreement with the Hide criterion
(eq. 17.1). Hysteresis occurs when one can find different
regimes for the same control parameters where the param-
eters have been approached from different directions that
is an increasing or decreasing Taylor number. Hystere-
sis is a well-known phenomenon for annulus experiments
and can generically be observed in free-surface [
Sitte and
Egbers
, 2000;
von Larcher and Egbers
, 2005b] and rigid
lid experiments [
Cole
, 1971;
Hignett et al.
, 1985]. For our
experimental setup a rather broad region of hysteresis
exists at the transition between
m
=3and
m
=4.The
existence of hysteresis depends not only on Ta, Ro, and Pr
but also on
η
and
[
von Larcher and Egbers
, 2005b].
Moreover, complex vacillating flow patterns are found
in the transition from axisymmetric flow to the wavy
flow regime (see Figure 17.3, upper row), where mode
Ta
<
1.5
×
17.3. RESULTS
Multivariate statistical techniques are suitable to under-
stand better the variability of the heated rotating flow.
We consider here four different methods: EOF analysis,
MSSA, POP analysis, and SV analysis. It is worth to
briefly mention the field of application of the different
techniques. This enables the reader to assess which tech-
nique is good for what purpose. For many applications it
is useful to decompose noisy multivariate data sets into
subsets or subspaces. A few patterns might span the “sig-
nal subspace” where the noise is captured in the “noise
subspace.” The specification of relevant patterns can be
done in many different ways, ranging from eigenmodes
(e.g., in terms of Bessel and trigonometric functions for
cylindrical geometry) to patterns that optimize certain
statistical moments [
H. von Storch
, 1995].
EOFs are defined as those patterns that are powerful
in explaining variance and thus the EOF method is the
method of choice for analyzing the variability of fields.
It is therefore widely used in the geosciences [
Lorenz
,
1956;
von Storch and Zwiers
, 1999]. This method also goes
by different names, e.g., principal component analysis or
proper orthogonal decomposition. The EOF method is
able to find the spatial patterns of variability and their
time variation and provides a measure for the relevance of
each pattern. Simply speaking, the EOF method breaks
the data into modes of variability that might (as is the
case for our data) be interpreted as physical modes of the
system. Strikingly, it can be shown that the EOF method
provides the most efficient way of capturing the dominant
components of a high-dimensional process with often
surprisingly few modes [
Holmes et al.
, 1996]. However,
more appropriate in our context is the use of the com-
plex EOF (CEOF) analysis [
Pfeffer et al.
, 1990;
von Storch
4(SV)
↓
3 (SV)
3
Tr
ansition to
irr
egular lo
w
as
indicated b
y
vi
sualization
2/3I
Ta
c
= 6.33×10
6
m=0
Ta
c
3× 10
6
10
7
10
8
3×10
8
Taylor number Ta
Figure 17.2.
Regime diagram observed for
η
= 0.38,
= 1.8,
and
T
=7.5K[
von Larcher and Egbers
, 2005b].
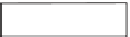




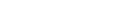


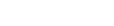

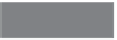


































































































































