Information Technology Reference
In-Depth Information
Figure 6. Comparison of ROC curves
many varIaBles In large samPles
There can be hundreds if not thousands of variables collected for each patient. There can be far too many
to include in any predictive model. We want to include all those variables that are crucial to the analysis,
including potential confounders, but the use of too many variables can cause the model to over-fit the
results, inflating the outcomes. Therefore, there needs to be some type of variable reduction method. In
the past, factor analysis has been used to reduce the set of variables prior to modeling the data. However,
there is now a more novel method available (Figure 7).
In our example, there are many additional variables that can be considered in this analysis. Therefore,
we use the variable selection technique to choose the most relevant. We first use the decision tree fol-
lowed by regression, and then regression followed by the decision tree.
Using the decision tree to define the variables, Figure 8 shows the ones that remain for the modeling.
Note that age, charges, and length of stay are at the beginning of the tree.
Figure 7. Variable selection

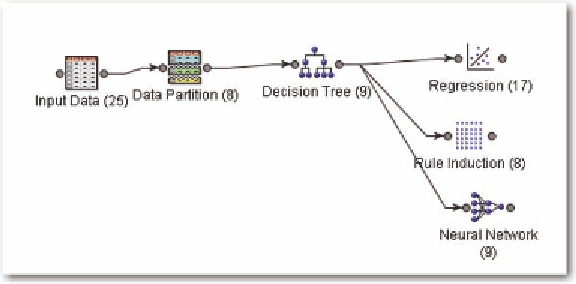
Search WWH ::

Custom Search