Image Processing Reference
In-Depth Information
The membership matrix will follow Equation 7.14 using hypertangent ker-
nel, but hesitation degree is incorporated into each membership degree.
Kernel-based IFCM clustering is robust on noise. It works well in a noisy
environment. Examples are shown on tumour images using all the three
kernels.
E x a mple 7. 5
Two examples are shown on CT scan images of the brain to show the
efficacy of the algorithm. Figures 7.7 and 7.8 show the tumour/clot in the
brain.
It is observed that simple intuitionistic fuzzy clustering does not per-
form better in a noisy environment but works well in a noisy environ-
ment. Radial basis kernel does not work well. Hypertangent kernel works
a little bit better than Gaussian kernel where the tumour/clot is clearly
detected.
(a)
(b)
(c)
(d)
(e)
FIGURE 7.7
(a) Brain clot image, (b) IFCM, (c) IFCM with Gaussian kernel, (d) IFCM with hypertangent ker-
nel and (e) IFCM with radial basis kernel. (Modified from Chaira, T. and Panwar, A.,
J. Comput.
Intell. Syst.
, 7(2), 1-11, 2013.)
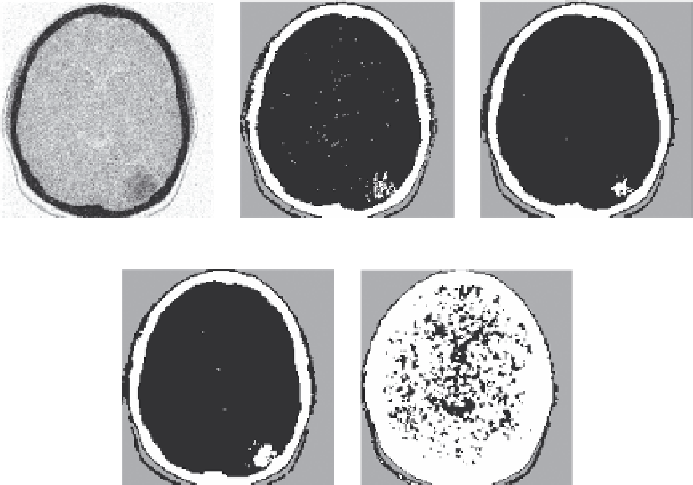
Search WWH ::

Custom Search