Geoscience Reference
In-Depth Information
The Spearman correlation results were 0.609, suggesting an increased estimate of accuracy
compared to the result from the contingency table assessment. Ji and Jensen (1999) also noted an
increase in accuracy when utilizing the rank correlation test. Of particular benefit would be subpixel
classifications that yield continuous data estimates as opposed to rank order data. These data would
allow for regression modeling that could be applied to the individual per-pixel errors.
The results presented here would have greatly benefited from a more accurate reference data
set. The lack of driveways in the planimetric data set affected a large proportion of the pixels and
probably served to underreport the actual truth for any given pixel. Intuitively, we feel that this
“lack of truth” probably had a greater effect on the per-pixel assessments (error matrix) than on
either the Spearman correlation or the whole-area approaches. This probably explains a portion of
the low per-pixel accuracy. However, all three approaches have been affected by the inaccuracies
in the truth set. For example, using nonrandom techniques, we sampled 50 driveway areas to derive
a total driveway area in the subwatershed. Summing the driveway area to the previously compiled
planimetric impervious surface area increased the accuracy of the whole-area approach to approx-
imately 85%. This underscores the need for high-quality reference data when assessing subpixel
estimates. However, reference data sets in the “real world” will always contain a certain proportion
of error. The GIS overlay framework effectively extrapolated the reference impervious surface to
correspond to the classified 30-m Dead Run cell. These spatial overlay methods provided here may
be repeated over any region to assess the accuracy of any pixel-based product.
The overlay framework also allows for the analysis of the spatial distribution of errors. Figure
19.3 is an error grid showing the absolute error per 30-m cell for the entire Dead Run subwatershed.
A cursory review of the error grid reveals that approximately 66% of the errors exist within the
1-20% (absolute error) range, signifying that a majority of cells (within the two data sets) are in
close agreement. This explains why the correlation assessment outperformed the assessment from
the contingency matrix. We can also discern that the contiguous blocks of error in the 90-100%
range are primarily due to areas not compiled in the truth data but present in the Landsat data. This
would include anthropogenic areas of interest such as parking lots as well as bare soil areas not
included in the truth data. Generally, in these contiguous areas of large error, the subpixel classi-
fication outperformed the truth data. We feel that this is in part due to a temporal disconnection
between the two data sets. Areas that were not included in the truth data set as of the date of the
imagery acquisition were actually present and imaged by the sensor. Misregistration between the
two data sets can also be observed in the error grid. Linear patterns appear to be associated with
the large roadways that traverse the area and are generally associated with the middle range of
error (30-80%). Spatial aggregation of error also contributes to decreased measures of accuracy
when using sample blocks of pixels compared to a simple random sampling scheme.
19.4 CONCLUSIONS
Results indicate that accuracy assessments of subpixel derived estimates based on per-pixel
sampling strategies may underestimate the overall accuracy of the map product. We believe this is
because per-pixel assessments of subpixel estimates are sensitive to registration accuracies, the
accuracy of the truth data, and classification variability at the pixel scale. A more robust subpixel
assessment may be achieved by applying whole-area (aggregate) or correlation-based approaches.
These approaches are less sensitive to differences in image registrations as well as errors in the
truth set and, in certain large-area applications such as watersheds, are probably a more realistic
indicator of the subpixel classification map accuracy.
With respect to impervious surfaces, we believe this technique has considerable merit when
considering water quality and watershed runoff models that require, as an input, the percentage of
impervious surface area above a given gauge or “pour point.” Our analysis shows that whole-area
scale estimates of a subpixel derived MOI can be relatively accurate even when the per-pixel
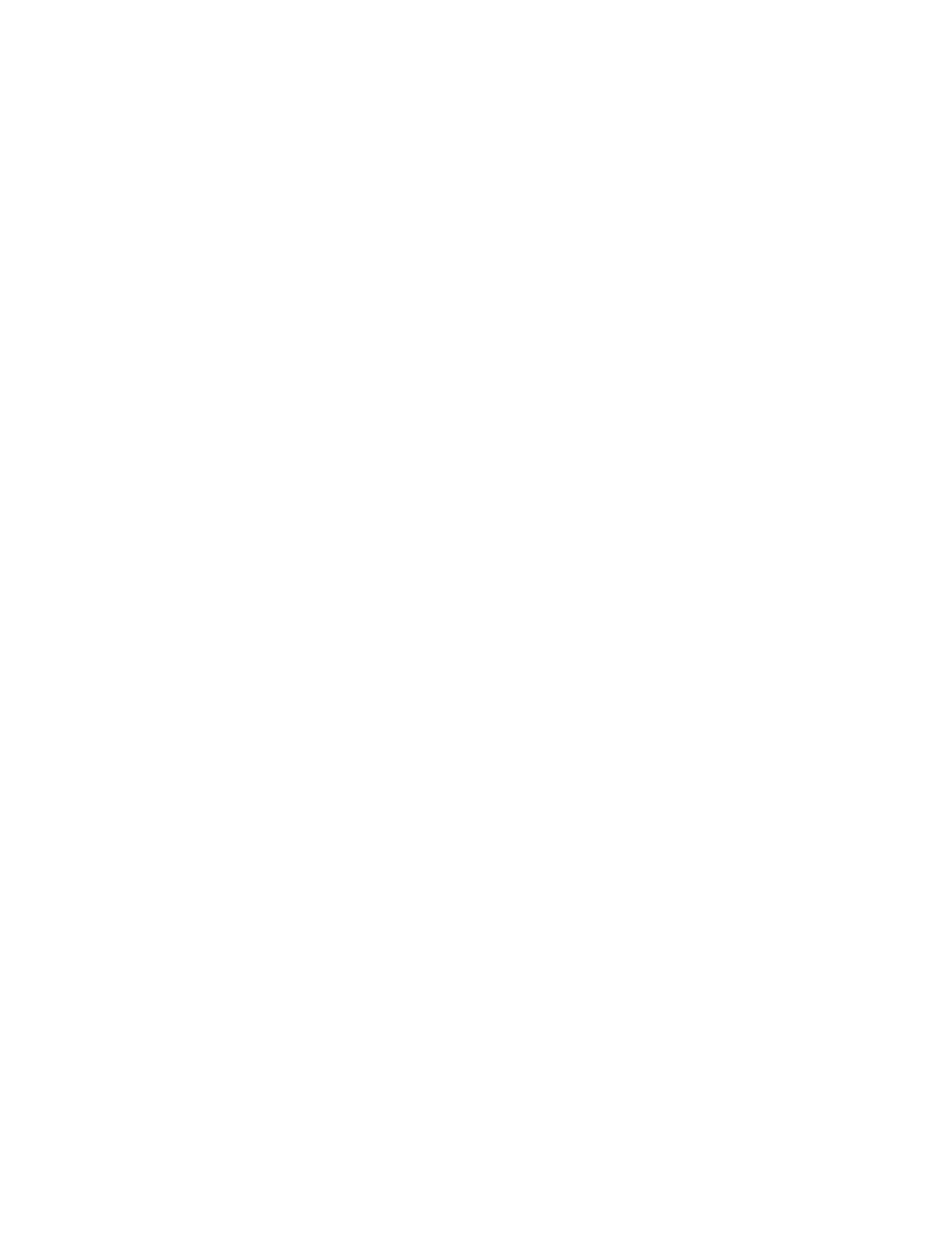














