Geoscience Reference
In-Depth Information
OK Errors
Errors
Number of Data 200
m ean -.0580
std. dev. 3.0722
coef. of var 307223.8000
maximum9.5430
upper quartile .9020
median .2880
lower quartile -.2110
minimum-24.2890
99.99
.250
99.9
99.8
99
98
.200
95
90
80
70
.150
5
60
40
30
20
.100
10
5
2
.050
1
.
.2
.000
.01
-8.0
-6.0
-4.0
-2.0
0.
2.0
4.0
6.0
8.0
-5.0
-3.0
-1.0
1.0
3.0
5.0
Variable
Variable
Errors versus True
True versus estimate
20.0
10.0
16.0
6.0
12.0
2.0
8.0
-2.0
4.0
-6.0
.0
-10.0
.0
4.0
8.0
12.0
16.0
20.0
.0
4.0
8.0
12.0
16.0
20.0
Estimate
True
Fig. 11.1
Error checks. Distribution of errors, cumulative distribution function of, True vs. Estimate, Error vs. True
should be ignored in the jackknifing process. Since the var-
iogram models are not very sensitive to small changes (see
comments regarding the robustness of kriging with respect to
variogram models, Chaps. 6 and 8), the error statistics may
be misleading or too similar to provide effective guidance.
Still, the technique may be useful as an indication of
how appropriate the variogram models are, in particular
when comparing two very different variogram models.
Also, the statistics generated are an aid in understanding
the results of a geostatistical analysis, and hence it should
be seen as another exploratory data analysis tool. It may
also detect serious modeling errors and flag numerical or
computational problems encountered when solving the
kriging equations.
The cross validation method based on splitting the data-
base in two subsets of the drill hole data is a more interesting
alternative as long as the domain is stationarity and there is
sufficient data to obtain a statistically meaningful set of errors.
This cross validation method has the same purpose explained
above. Journel and Rossi (
1989
) describe a case where this
cross validation was used to evaluate the differences between
ordinary and universal kriging. If a “true” dataset is available,
then the results can be compared in terms of distribution of
errors, as shown in Fig.
11.1
. These comparisons can be show
as distribution of the errors (PDF and CDFs) and a cross plot
of true vs. estimated values. Also it is useful to look at the
relationship between errors and true values.
The magnitude of the errors may be correlated to location,
and thus is it useful to obtain a location map of errors, as
shown in Fig.
11.2
. Cross-validation can thus be considered
a rehearsal before the production run.
11.4
Resource Model Validation
The mineral resource model should be validated using statis-
tical and graphical tools (Leuangthong et al.
2004
). The sug-
gested checks are useful to ensure the internal consistency
of the model, which implies that the model has the expected
characteristics; also, that there are no gross or spurious er-
rors, and that all processes were implemented correctly. The
resource model validation in fact should cover the data and
the geological model used, the estimation domains defined,
and the geostatistical model applied.
It is good practice to allow for model iterations in the
model building schedule and budget. By changing estimation
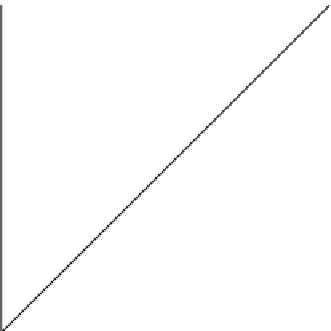





































































































































































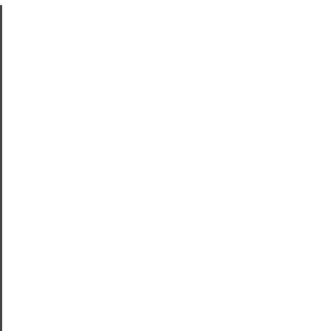




























































































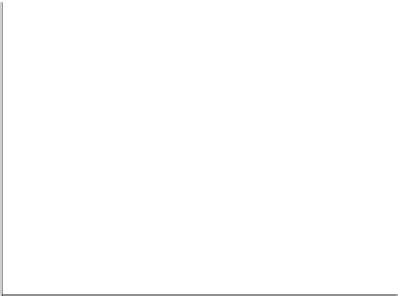






























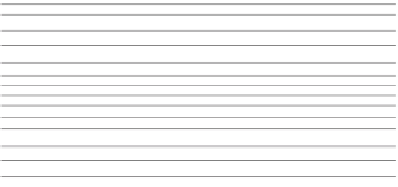

