Biomedical Engineering Reference
In-Depth Information
100%
95%
90%
85%
80%
75%
70%
65%
60%
55%
50%
Binary Model
Multi-Label Model
Simple Sum Rule
Decision Fusion Method
Figure 11.22:
Recognition rates (foreground only) of multiple classifier systems
based on binary performance model, multilabel performance model, and simple
sun fusion.
tation. These a posteriori performances (conceptually equivalent to the
recog-
nition rate
of the classifier) were then plotted versus the estimates computed
by either of the EM methods. It is easy to see that there is a very good agree-
ment between the actual and the the estimated parameters, with a slightly higher
predictive accuracy following the binary performance model. The Pearson cor-
relation coefficient between true and estimated performances was 0
.
94 for the
binary expert model, and 0
.
87 for the multilabel expert model. The increased
quality of the parameter estimation using the binary performance model can
be explained by the substantially larger number of degrees of freedom in the
multilabel model, due to the interlabel crosstalk coefficients.
As Fig. 11.22 illustrates, the accuracy of a multiclassifier segmentation can
be improved considerably when the performance parameters of the individual
classifiers are estimated and taken into account. Overall, the estimation method
using a multilabel performance parameter model was slightly less accurate in
estimating the actual parameters, but produced a slightly better segmentation
accuracy than the method based on a binary performance model.
11.7
Conclusion
This chapter has shed light on some often overlooked aspects of atlas-based
segmentation methods. We have compared four different strategies for atlas
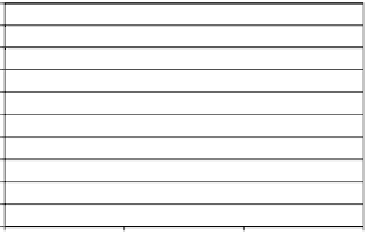





