Information Technology Reference
In-Depth Information
the network is trained, its final parameter values are stored and the network is used
for prediction. In this experiment, the first 200 data samples were used for training
and the remaining 200 data samples were used for evaluation. The training
performance of the network is illustrated in Figure 6.9(b) and also listed in Table
6.3(a). It is also illustrated that using only
M
= 10 fuzzy rules (
first model
) and also
10 Gaussian membership functions implemented for fuzzy partition of the input
universe of discourse the proposed training algorithm could bring the network
performance index (SSE) down to 3.0836 × 10
-4
or equivalently MSE to 3.0836 ×
10
-6
from their initial values 45.338 in only 999 epochs. This is equivalent to
achieving an actual SSE = 0.0012 or an actual MSE = 1.1866 × 10
-5
when
computed back on the original data.
u(k ), g
(k
) a nd y(k)
p
lo t o f W a n
g
d a ta
2
1
0
-1
-2
0
50
100
150
200
250
300
350
400
1
0.5
0
-0 .5
-1
0
50
100
150
200
250
300
350
400
2
1
0
-1
-2
0
50
100
150
200
250
300
350
400
Figure 6.9(a).
Plot of first input
u
(
k
) (top), output
g
(
k
) (middle) and second input
y
(
k
)
(bottom) of non-scaled Wang data (second-order nonlinear plant).
The corresponding evaluation performance of the trained network, as shown in
Figure 6.9(c) and also listed in Table 6.3(a), illustrates that using the scaled or
normalized evaluation data set from 201 to 400, the SSE value of 5.5319 × 10
-4
, or
equivalently MSE value of 5.5319 × 10
-6
, were obtained. The above results further
correspond to an actual SSE value of 0.0021, or equivalently to an actual MSE
value of 2.1268 × 10
-5
, which were computed back on the original evaluation data
set. Evidently, the evaluation performance (actual MSE value), reported in Table
6.3(b), is at least 10 times better than that achieved by Setnes and Roubos (2000),
Roubos and Setnes (2001) and much better than that achieved by Yen and Wang
(1998, 1999a, 1999b).
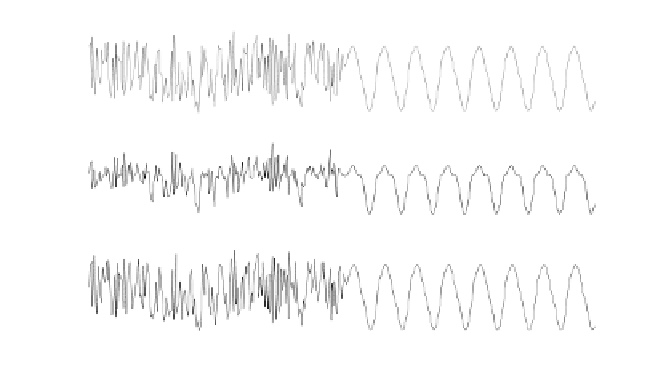

























































































































































Search WWH ::

Custom Search