Information Technology Reference
In-Depth Information
Concrete/Abstract Semantics
developing at the same time as the mappings are being
learned (see section 10.6 for a model of the develop-
ment of semantic representations). Furthermore, people
usually learn the semantic and phonological representa-
tions for words before they learn the corresponding or-
thography (and illiterate people never learn the orthog-
raphy). Thus, the model does not accurately capture
many aspects of language acquisition. Nevertheless, the
trained model does appear to provide a reasonable ap-
proximation to the distributed nature of word represen-
tations in a literate adult.
Twenty of the 40 words in our training corpus were
concrete
nouns (i.e., referring to physical objects), and
20 were more
abstract
. As mentioned above, people
with deep dyslexia treat these two types of words dif-
ferently. Specifically, they make more semantic errors
on abstract words than concrete ones, presumably be-
cause the semantic representations of concrete words
are richer and more robust to damage. The two sets of
words were closely matched on orthographic features
(table 10.3).
The semantic representations developed by Plaut and
Shallice (1993) capture the concrete/abstract distinction
by having different numbers of semantic feature units.
There are 67 such features for the concrete words (e.g.,
main-shape-3d
,
found-woods
,
living
), and 31 for the ab-
stract ones (e.g.,
has-duration
,
relates-location
,
quality-
difficulty
). The concrete words have an average of 18.05
features active per word, while the abstract words have
an average of only 4.95 features active. These are ob-
viously “cartoon” semantics that fail to capture the full
subtlety of the real thing, but the similarity in activa-
tion patterns between words does a reasonable job of
capturing general intuitions, as shown in a cluster plot
(figure 10.7). We retain the distinction between con-
crete and abstract words in the semantic hidden layers
by dividing the units into two groups, corresponding to
concrete semantic features (35 units) and abstract se-
mantic features (21 units).
We slightly modified the Plaut and Shallice (1993)
representations of orthography and phonology for our
model, adopting consistent, simple representations for
both. These simplifications are appropriate for the sim-
plified, small-scale nature of this model; subsequent
models will use more realistic representations.
Y
40.00
flag
coat
star
case
lock
35.00
post
rope
wage
cost
stay
hir
loa
rent
30.00
role
fact
loss
25.00
gain
ease
past
tact
deed
20.00
need
lack
plea
hin
pla
flaw
15.00
flan
tart
hind
10.00
loon
har
deer
reed
gri
wave
5.00
face
lass
flea
tent
0.00
X
0.00
10.00
20.00
30.00
40.00
Figure 10.7:
Cluster plot of the similarity of the semantic
representations for the 40 different words, where the abstract
words occupy the central cluster, surrounded by two main
branches of the concrete words, which correspond roughly to
living versus synthetic things.
Plaut and Shallice (1993) orthographic representations
were distributed at both the word and letter level. We
eliminated the letter-level distributedness by using indi-
vidual units to represent a letter, but retained the word-
level distributedness by constructing words as combi-
nations of letter-level activations across 4 slots in left-
to-right order. The letter-level distributed features were
not actually used for any relevant aspect of the model,
and the localist letter units are more consistent with the
original phonological representations.
The Plaut and Shallice (1993) phonological repre-
sentations had 7 slots of localist phoneme units, with
“blank” units for unused slots. We instead use our
standard repeating phonology representations described
previously in section 10.2.2, which are consistent with
those used for subsequent simulations. However, in-
stead of using distributed features for each phoneme
(as we do in subsequent models), we just used individ-
ual units to represent each phoneme (as in the original
model).
The
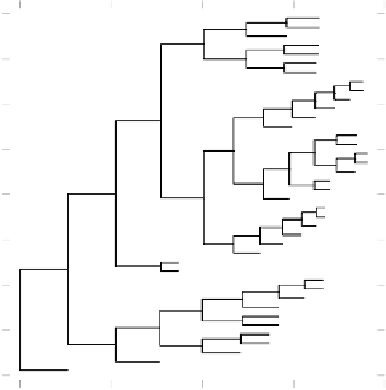
Search WWH ::

Custom Search