Information Technology Reference
In-Depth Information
Semantics
Conc Phon Abst Phon
tart tttartt tact ttt@ktt
tent tttentt rent rrrentt
face fffAsss fact fff@ktt
deer dddErrr deed dddEddd
coat kkkOttt cost kkkostt
grin grrinnn gain gggAnnn
lock lllakkk lack lll@kkk
rope rrrOppp role rrrOlll
hare hhhArrr hire hhhIrrr
lass lll@sss loss lllosss
flan fllonnn plan pll@nnn
hind hhhIndd hint hhhintt
wave wwwAvvv wage wwwAjjj
flea fllE--- plea pllE---
star sttarrr stay sttA---
reed rrrEddd need nnnEddd
loon lllUnnn loan lllOnnn
case kkkAsss ease ---Ezzz
flag fll@ggg flaw fllo---
post pppOstt past ppp@stt
OS_Hid
SP_Hid
w
st
pr
l n
hk
fg
−d
z
tv
rs
np
kl
gj
−d
z
tv
rs
np
kl
gj
−d
z
tv
rs
np
kl
gj
−d
twy
adegknr s
i l nopr sv
l or t aceg
pr st waei
cdef ghl n
tw
pr
l n
hk
fg
−d
tw
pr
l n
hk
fg
−d
i o
ae
OU
EI
@A
Orthography
OP_Hid
Phonology
Figure 10.6:
The model, having full bidirectional connectiv-
ity between orthography, phonology, and semantics.
Table 10.3:
Words used in the simulation, with 20 concrete
and 20 abstract words roughly matched on orthographic simi-
larity. Also shown is the phonological representation for each
word.
10.3.4
Basic Properties of the Model
Our model is based directly on one developed to sim-
ulate deep dyslexia (Plaut & Shallice, 1993; Plaut,
1995). We use the same set of words and roughly the
same representations for these words, as described be-
low. However, the original model had only the path-
ways from orthography to semantics to phonology, but
not the direct pathway from orthography to phonology.
This pathway in the original model was assumed to be
completely lesioned in deep dyslexia; further damage
to various other parts of the original model allowed
it to simulate the effects seen in deep dyslexia. Our
model includes the direct pathway, so we are able to
explore a wider range of phenomena, including surface
and phonological dyslexia, and a possibly simpler ac-
count of deep dyslexia.
Our model looks essentially identical to figure 10.5,
and is shown in figure 10.6. There are 49 hidden units
in the direct pathway hidden layer (
OP_Hid
), and 56
in each of the semantic pathway hidden layers. Plaut
and Shallice (1993) emphasized the importance of
at-
tractor dynamics
(chapter 3) to encourage the network
to settle into one of the trained patterns, thereby pro-
viding a
cleanup
mechanism that cleans up an initially
“messy” pattern into a known one. This mechanism re-
duces the number of “blend” responses containing com-
ponents from different words. We implemented this
cleanup idea by providing each of the main representa-
tional layers (orthography, semantics, and phonology)
with a recurrent self-connection.
To train the network, we used largely the same repre-
sentations developed by Plaut and Shallice (1993) for
the orthography, phonology, and semantics of a set of
40 words, and taught the network to associate the corre-
sponding representations for each word. Thus, we seem
to be somewhat implausibly assuming that the language
learning task amounts to just associating existing rep-
resentations of each type (orthography, phonology, and
semantics).
In reality, these representations are likely
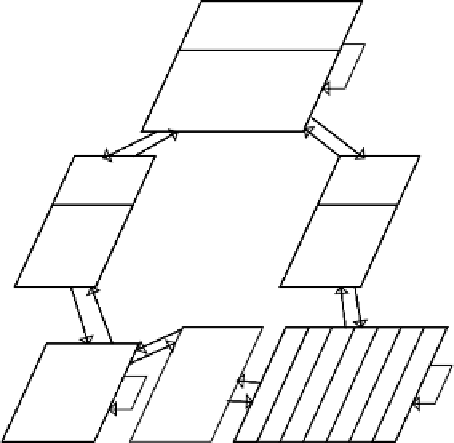


Search WWH ::

Custom Search