Image Processing Reference
In-Depth Information
Figure 18.9c
. This experiment indicates that the ST-SVR method could meet
the need for sensitive and robust fMRI signal analysis.
18.5
CONCLUSIONS AND DISCUSSIONS
From a signal processing viewpoint, fMRI activation detection is a problem
of nonlinear spatiotemporal system identification. We have presented a novel
regression model involving spatiotemporal correlations using SVR, where
nonlinear signal analysis is achieved. The framework allows the merging of
model-driven with data-driven methods, as well as multiresolution signal anal-
ysis, by adjusting the associated model and scale parameters. Other advantages
of the approach are embedded removal of low-frequency noise components
and easy incorporation of multirun and multisubject studies into the frame-
work. Experimental results on both simulated and real fMRI data revealed its
effectiveness. Some comments on the particulars of the method are discussed
in the following text.
A simple way to understand SVR is to consider it as a device that converts
a highly nonlinear regression problem into a linear one by expanding the input
variables using a series of nonlinear functions. This is closely related to the use
of multiple basis functions of peristimulus time used in conventional analyses of
fMRI time series. Another ubiquitous example is the use of discreet cosine
functions of time that model low-frequency drift terms of smooth but arbitrary
form. SVR goes further than simply expanding each component of the input. It
also explicitly includes high-order interactions among the inputs. An analogy here
might be the use of Volterra series expansions that use the same device to estimate
high-order kernels (15) that model interactions among stimuli used in producing
fMRI responses. These examples are used to provide an intuition into how SVR
can model arbitrary and complicated nonlinear relationships between explanatory
and response variables.
The size of the spatial window within which the SVR is performed is set to
3
1. We choose this size to allow for some spatial continuity
while limiting the likelihood of heterogeneous activation within the same window.
In addition, the window is intended to cover a brain region whose physical size
is approximately isotropic. Alternatively, a Gaussian window can be applied to
achieve more continuous weighting.
Correction for head motion involves rigid-body transformation estimation
and resampling. In the multisubject case, the raw data need to be aligned through
nonrigid transformation and resampling. Correcting differences in slice timing of
2-D acquisitions also needs data resampling. Because of the thick image slices
typical of fMRI, intensity interpolation, required during the resampling process,
can introduce significant artifacts (19). With the proposed ST-SVR approach,
continuous variables are used for both the input vectors and output scalar. So,
given the estimated motion parameters, nonrigid transformation parameters, and
slice-timing differences, we could directly keep and use the floating-point con-
tinuous values for the spatial coordinates and time indices in the ST-SVR learning.
×
3
×
3 or 3
×
3
×
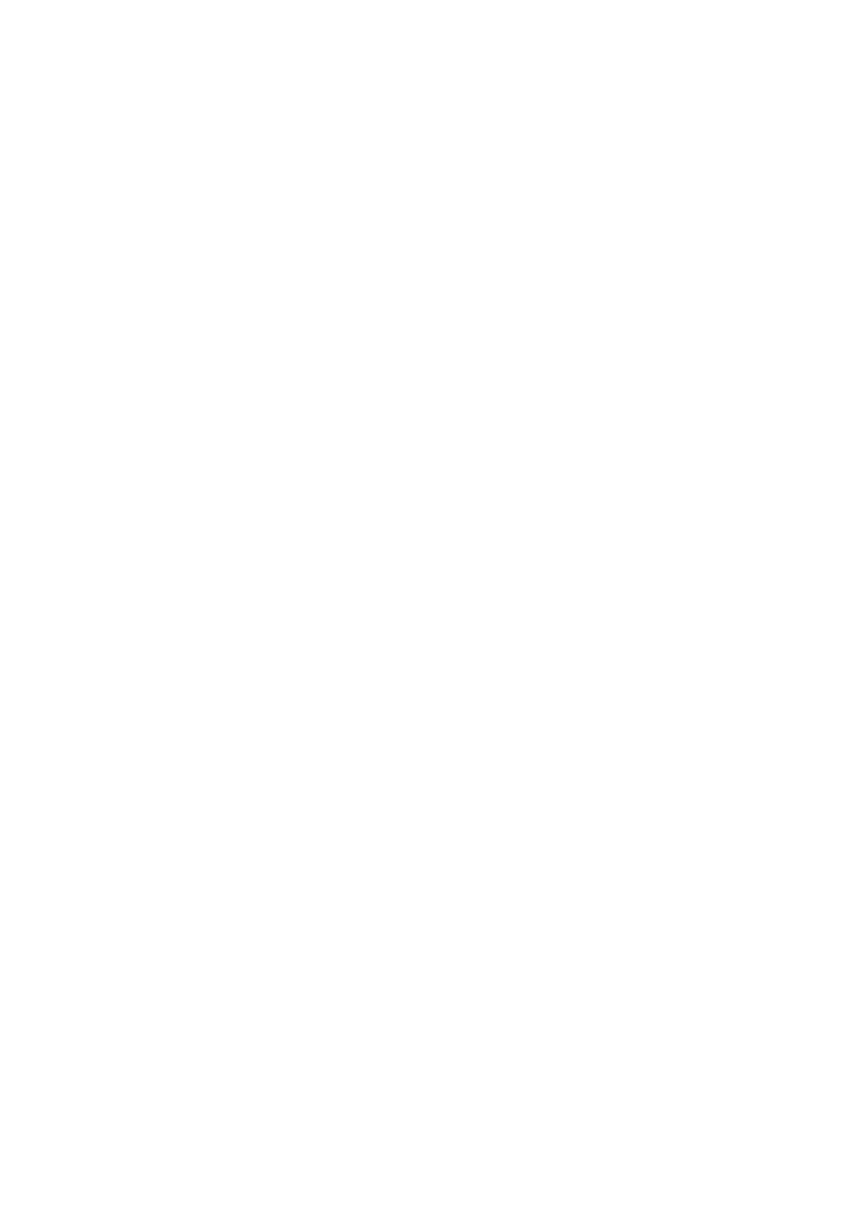





































Search WWH ::

Custom Search