Image Processing Reference
In-Depth Information
reasonable lattice representation of an underlying and smooth Gaussian
field. This necessitates smoothness to be substantially greater than
voxel size. If the voxels are large, then they can be reduced by sub-
sampling the data and smoothing (with the original point spread func-
tion) with little loss of intrinsic resolution.
4.
In the context of intersubject averaging it is often necessary to smooth
more (e.g., 8 to 12 mm) to project the data onto a spatial scale where
homologies in functional anatomy are expressed among subjects.
17.3
GENERAL LINEAR MODEL
Statistical analysis of fMRI data entails (1) modeling the data to partition observed
neurophysiological responses into components of interest, confounds, and error,
and (2) making inferences about the interesting effects in relation to the error
variance. This can be regarded as a direct comparison of the variance attributable
to an interesting experimental manipulation to the variance attributable to the
error. These comparisons can be made with T or F statistics.
A brief review of the literature may give the impression that there are numerous
ways to analyze fMRI time series with a diversity of statistical and conceptual
approaches. This is, however, not the case. With few exceptions, every analysis is a
variant of the general linear model (GLM). This includes: (1) simple T tests on scans
assigned to one condition or another, (2) correlation coefficients between observed
responses and boxcar stimulus functions in fMRI, (3) inferences made using multiple
linear regression, (4) evoked responses estimated using linear time invariant models,
and (5) selective averaging to estimate event-related responses. Mathematically, they
are formally identical and can be implemented with the same equations and algo-
rithms. The only thing that distinguishes among them is the design matrix encoding
the experimental design. The use of the correlation coefficient deserves special
mention because of its popularity in fMRI (11). The significance of a correlation is
identical to the significance of the equivalent T statistic testing for a regression of
the data on the stimulus function. The correlation coefficient approach is useful but
the inference is effectively based on a limiting case of multiple linear regression that
obtains when there is only one regressor. In fMRI, many regressors usually enter
into a statistical model. Therefore, the T statistic provides a more versatile and generic
way of assessing the significance of regional effects.
17.3.1
D
M
ESIGN
ATRIX
The GLM is an equation
Y
=
X
β
+
ε
that expresses the observed response variable
Y
plus a well-behaved
error term. The GLM is variously known as “analysis of covariance” or “multiple
regression analysis” and subsumes simpler variants like the “T test” for a differ-
ence in means to more elaborate linear convolution models such as finite impulse
response (FIR) models. The matrix
in terms of a linear combination of explanatory variables
X
that contains the explanatory variables (e.g.,
designed effects or confounds) is called the
X
design matrix
. Each column of the
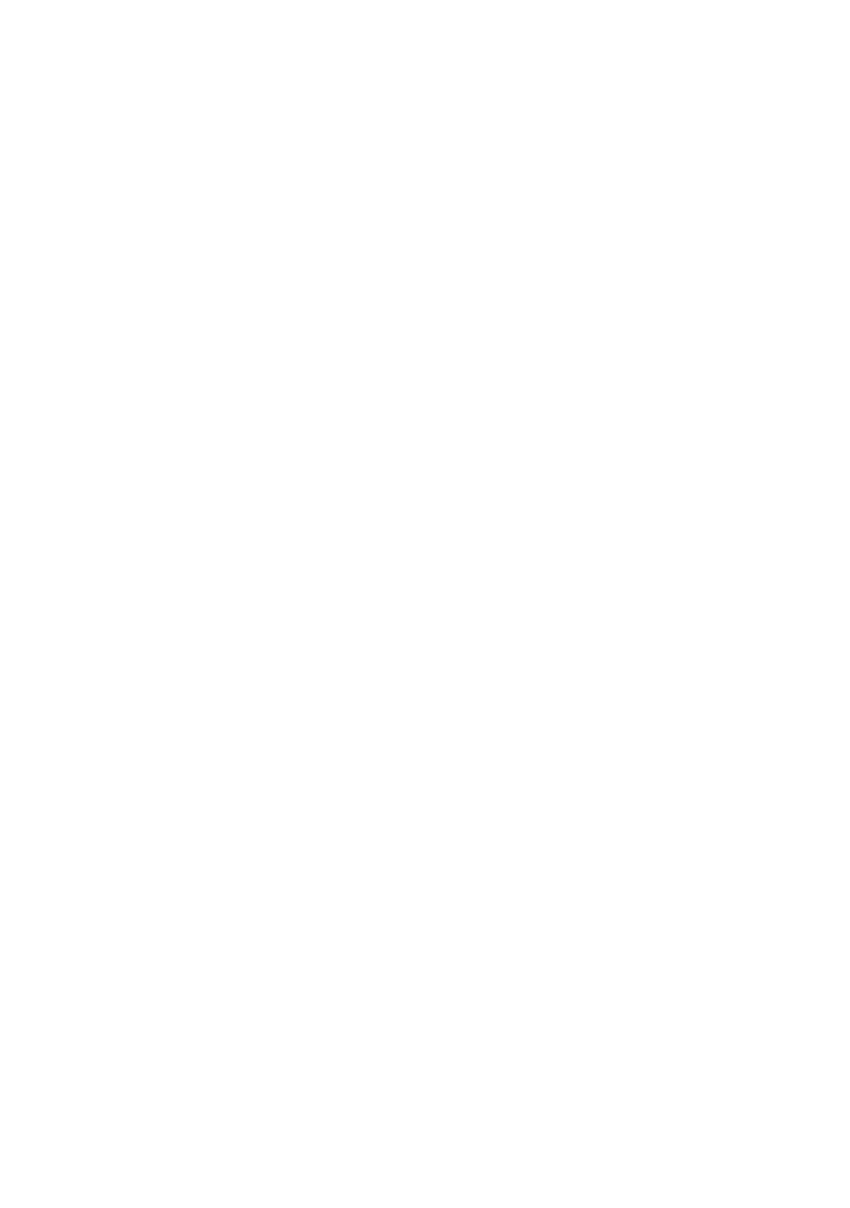





































Search WWH ::

Custom Search