Image Processing Reference
In-Depth Information
FIGURE 17.2
Schematic illustrating a Gauss-Newton scheme for maximizing the pos-
terior probability
) of the parameters required to spatially normalize an image. This
scheme is iterative. At each step the conditional estimate of the parameters is obtained by
jointly minimizing the likelihood and prior potentials. The former is the difference between
a resampled (i.e., warped) version
p
(
θ
|
y
y
of the image
f
and the best linear combination of
some templates
. These parameters are used to mix the templates and resample the image
to progressively reduce both the spatial and intensity differences. After convergence the
resampled image can be considered normalized.
g
schemes have been proposed to correct for the distortion effects (10). However,
this distortion is not an issue if one spatially normalizes the functional data.
17.2.5
S
S
PATIAL
MOOTHING
The motivations for smoothing the data are fourfold:
1.
By the matched filter theorem, the optimum smoothing kernel corre-
sponds to the size of the effect that one anticipates. The spatial scale
of hemodynamic responses is, according to high-resolution optical
imaging experiments, about 2 to 5 mm. Despite the potentially high
resolution afforded by fMRI an equivalent smoothing is suggested for
most applications.
2.
By the central limit theorem, smoothing the data will render the errors
more normal in their distribution and ensure the validity of inferences
based on parametric tests.
3.
When making inferences about regional effects using GRF theory (see
the following subsection), the assumption is that the error terms are a
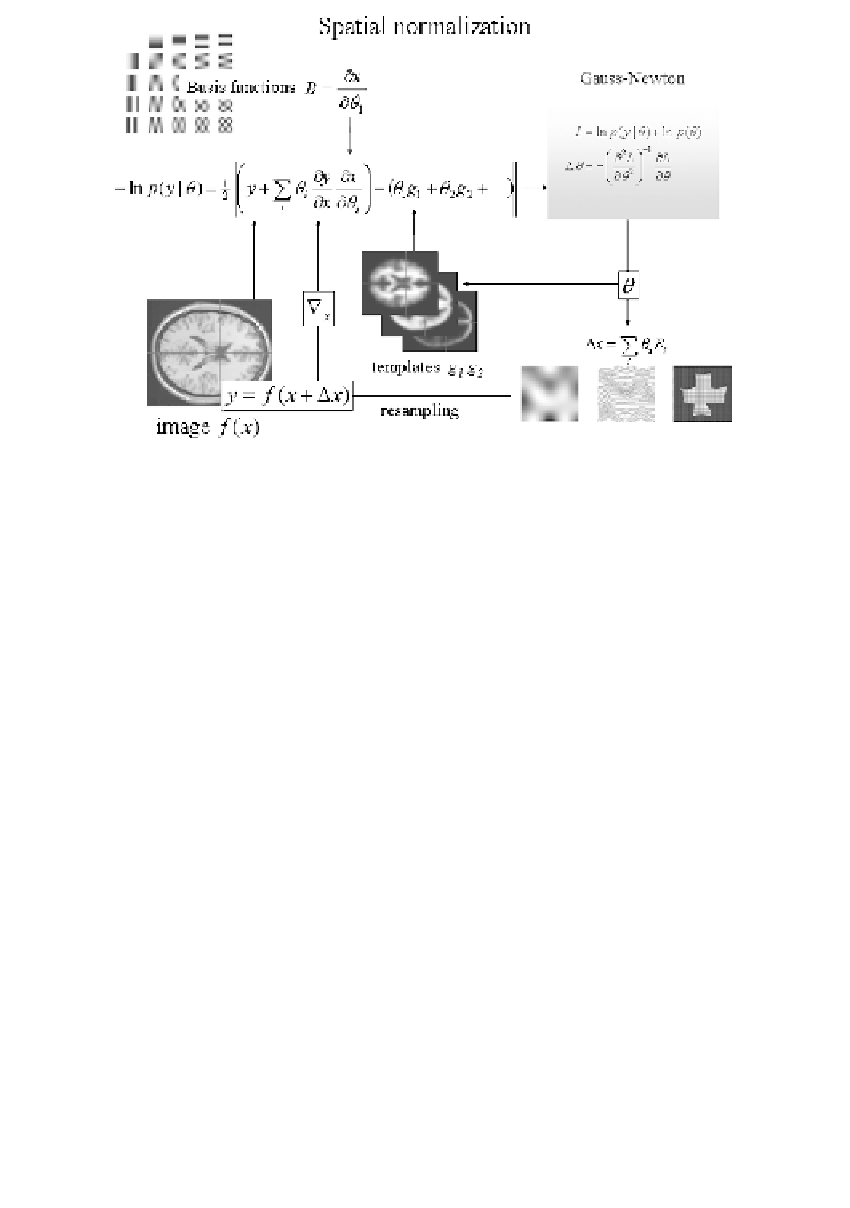





































Search WWH ::

Custom Search