Information Technology Reference
In-Depth Information
ln
N
−
ln
η
R
(
ω
I
)
−
R
emp
(
ω
I
)
≤
,
(27)
2
I
−
ln
η
R
emp
(
ω
0
)
−
R
(
ω
0
)
≤
.
(28)
2
I
And since, by definition of
ω
I
)
, the (26) follows.
Going back to the cross-validation procedure, we notice that in each single fold
the measure
R
emp
corresponds by analogy to the measure
R
in (26) and the measure
R
emp
corresponds by analogy to
R
emp
therein. Obviously
R
is defined on an infinite and
continuous space
Z
=
X
ω
I
,
R
emp
(
ω
0
)
≥
R
emp
(
×
Y
, whereas
R
emp
is defined on a discrete and finite sam-
ple
{
z
1
,...,
z
I
}
, but still from the perspective of a single cross-validation fold we may
I
)
as the “target” minimal
probability
of misclassification and
R
emp
(
view
R
emp
(
ω
I
)
as
the observed relative
frequency
of misclassification — an estimate of that
probability
,
remember that we take random subsets
ω
z
1
,...,
z
I
}
{
{
z
1
,...,
z
I
}
from the whole set
.
We write
ω
I
)+
−
ln
η
R
emp
(
R
emp
(
ω
I
)
≤
ω
I
)
≤
R
emp
(
.
(29)
2
I
The first inequality is true with probability 1 by definition of
ω
I
. The second is a Cher-
noff inequality, true with probability at least 1
.
Now, we plug (29) into (23) and obtain with probability 1
−
η
k
=
1
k
(
n
1
)
k
(
2
)
k
)
−
(
−
∑
−
η
−
η
or greater:
n
n
R
emp
(
ω
I
)+
1
−
ln
η
C
≤
2
I
+
ln
N
+
−
ln
η
−
ln
η
2
I
2
I
ln
N
ω
I
)+
n
n
−
ln
η
=
R
emp
(
−
1
2
I
+
√
n
+
n
n
−
ln
η
−
1
2
I
ω
I
)+
n
n
1
ln
N
−
ln
η
=
R
emp
(
1
+
1
−
−
2
I
1
+
√
n
+
n
n
−
ln
η
−
2
I
=
V
+
n
n
1
ln
N
−
ln
η
1
−
−
2
I
1
+
√
n
+
n
n
−
ln
η
.
−
2
I
This concludes the proof of theorem 2.










































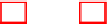