Environmental Engineering Reference
In-Depth Information
TABLE 16.3
Results of the OLS regression of mean NDVI rank.
TABLE 16.4
Results of the spatial lag and spatial error
regressions of mean NDVI rank.
Explanatory
Model 1
Model 2
Model 3
Explanatory variable
Spatial lag
Spatial error
variable
%African
model
model
−
0.44***
−
0.13***
0.02
% African American
0.04
−0.04
American
(−15.88)
(−4.44)
(0.66)
(0.03)
(0.05)
% Hispanic (ln)
−
0.22***
−
0.07*
0.00
% Hispanic (ln)
−
0.19
0.12
(
−
7.88)
(
−
2.55)
(0.18)
(0.39)
(0.41)
% High School
0.56***
0.45***
% High School
0.48***
0.65***
(18.12)
(16.01)
(0.07)
(0.10)
Population
−
0.44***
Population density
−
0.002***
−
0.003***
Density
(
−
16.76)
(0.0002)
(0.0003)
Constant
92.65***
−77.61***
−33.01***
Spatial lag term
0.75***
(0.02)
(57.60)
(-8.17)
(−3.76)
Adjusted
R
2
0.25
0.44
0.57
Spatial error term
0.86***
(0.02)
AIC
9911
9629
9384
Moran's
I
0.684***
0.575***
0.501***
Constant
−
15.85**
25.31 **
LM Lag
1329***
957***
726***
(5.81)
(9.77)
Robust LM Lag
95***
115***
108***
AIC
8705.17
8737.26
LM Error
1245 ***
880***
668***
Moran's
I
0.040*
0.005
Robust LM Error
10***
38***
50***
*
p
<
0
.
05,**
p
<
0
.
01, ***
p
<
0
.
005.
Reported values are unstandardized coefficients, with standard errors in
parentheses.
*
p
<
0
.
05,**
p
<
0
.
01, ***
p
<
0
.
005.
Reported values are standardized coefficients, with t-values in parentheses.
variable as an explanatory variable in the model, which may be
expressed as
(Table 16.3), though the value of the LM lag statistic is greater
than that of the LM error statistic.
Asthenatureandcauseofthespatialdependencyinthemodel
residuals is unknown, Table 16.4 reports the results of both the
spatial lag and spatial error forms of the spatial econometric
model. They are similar in that for both models percent high
school and population density are significant, and the race
variables are not. The spatial lag and spatial error terms in each
model are also significant. Spatial autocorrelation in the model
residuals, as indicated by Moran's
I
, persists slightly in the spatial
lag model but not in the spatial error model. Figure 16.2 presents
the Moran scatterplot (Anselin, 1996) for the OLS (Table 16.3,
model 3) and spatial error models. This figure presents the nature
of the spatial autocorrelation in the model residuals, where each
point represents the value of the model residual and the spatial
lag of the model residual for an observation, such that the higher
the slope of the line of best fit, the greater the degree of spatial
autocorrelation in the model residuals. Clearly, the slope of the
line of best fit is much lower for the spatial error model, and not
significantly different than zero, as compared to the OLS model.
The results of the case study suggest that African Americans
and Hispanics tend to live in places with less healthy green
vegetation than other people in the Philadelphia, Pennsylvania
metropolitan area. This relationship of race with vegetation is
partly explained by class, specifically educational attainment,
as highly educated people tend to live in greener areas. How-
ever, even after accounting for educational attainment, African
Americans and Hispanics still tend to live in less green areas of
y
=
ρWy
+
Xβ
+
ε
where
ρ
is the spatial autogregressive coefficient, and
W
is the
spatial weights matrix. Maximum likelihood is employed to
estimate the model (Anselin, 1988). Alternatively, the spatial
error form of the spatial econometric model allows the error
term to vary over space, such that
y
=
ρWu
+
Xβ
+
ε
where
ρWu
represents the spatially autocorrelated component
of the error term.
Generally, the spatial lag model is appropriate where one
hypothesizes that a causal process of diffusion that occurs across
space from one spatial unit to another is responsible for the
spatial autocorrelation in the residuals. The spatial error model
treats the spatial dependency as a nuisance to be accounted for
so that the assumptions of regression may be met and unbiased
parameter estimates may be obtained. Such spatial dependency
in the model residuals may be due to model misspecification in
the form of missing variables or nonlinear relationships among
explanatory and dependent variables. The Lagrange multiplier
(LM) statistics and their robust forms also offer some guidance
as to the appropriate form of the spatial econometric model to
adopt (Anselin
et al
., 1996). In the present study, LM statistics
for the both the lag and error models are highly significant















































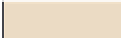




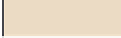




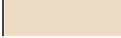
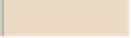
















































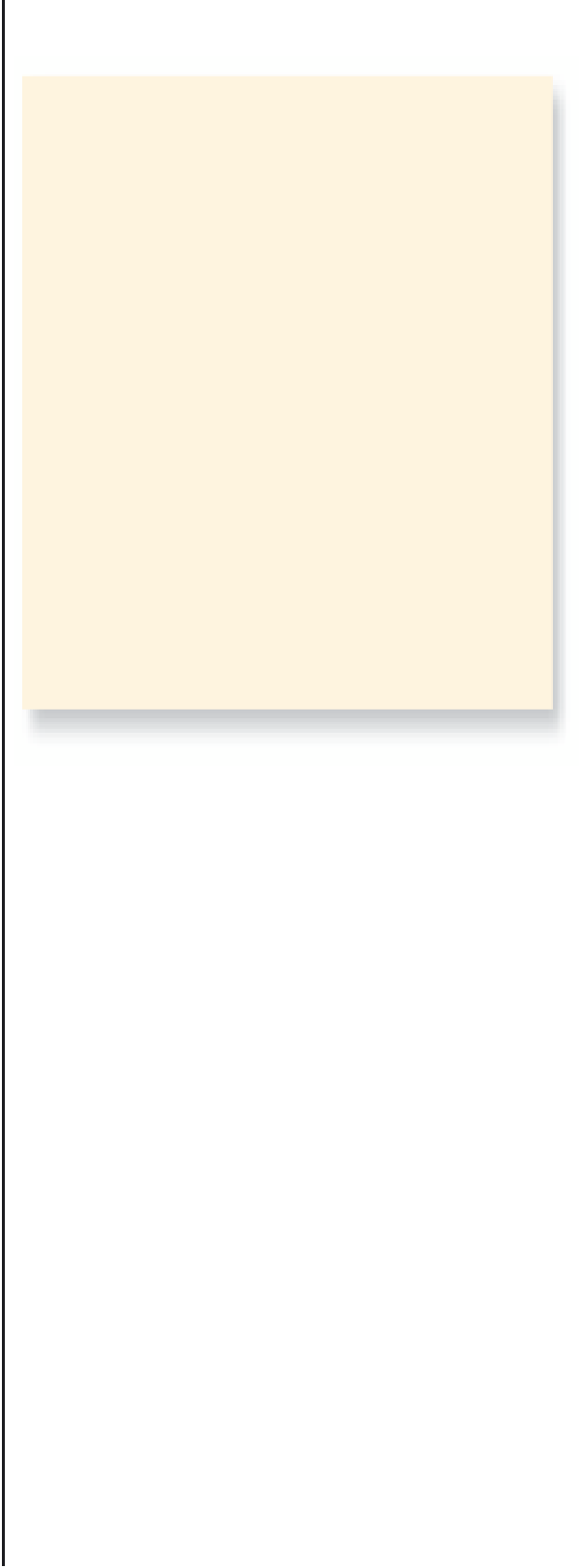














































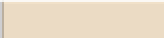


























Search WWH ::

Custom Search