Biomedical Engineering Reference
In-Depth Information
Fig. 3.16 Comparison of
average velocity estimation
of group number 1 for
Head_1
60
Measurement
Kalman Filter
IMME
MC-IMME
50
40
30
20
10
0
-10
-20
0.01
0.02
0.03
0.04
0.05
0.06
0.07
0.08
Data Time Index (sec)
Fig. 3.17 Comparison of the
velocity estimations with no
feedback/forward versus
feedback/forward
2
1
0
-1
-2
-3
-4
Measurement
No feedback/forward
feedback/forward
-5
-6
-7
0.03
0.04
0.05
0.06
0.07
0.08
0.09
0.1
Data Time Index(sec)
observed in Fig.
3.17
that the feedback/forward method slightly increases the
performance of pure MC-IMME in 14 %.
We show all nine datasets to compare the overall performance of velocity error
averaged among eight channels between no feedback/forward versus feedback/
forward. Table
3.4
shows the overall performance of velocity error among the
datasets listed in Table
3.2
. Given in Table
3.4
, feedback/forward method out-
performs
no
feedback/forward
method
around
15-37 %
for
Chest
dataset,
20-26 % for Head dataset, and 14-22 % for Upper Body dataset.
3.5.5 Prediction Overshoot
We define overshoot for cases in which the predicted output exceeds a certain
marginal value with confidence levels corresponding to the tolerances [
57
]. The
initialization process is an essential step of Kalman filter-based target tracking.
Unfortunately, this process produces an unexpected prediction estimate error. To
compensate for the prediction estimate error, we used a marginal value to generate a
95 % prediction interval for the measurement prediction, so that we can define the
upper bound and the lower bound by adding the marginal value to the measurement
and subtracting the marginal value from the measurement, respectively [
57
].
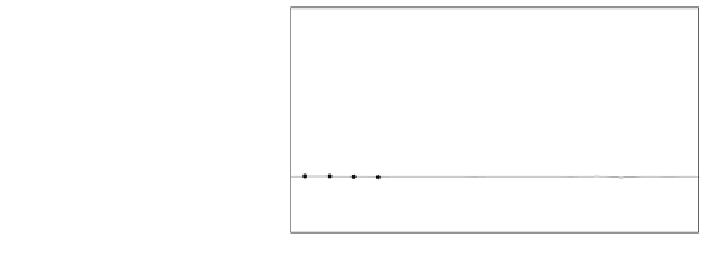


























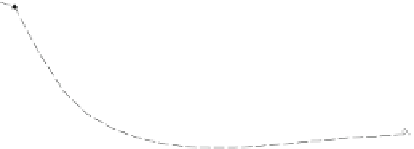















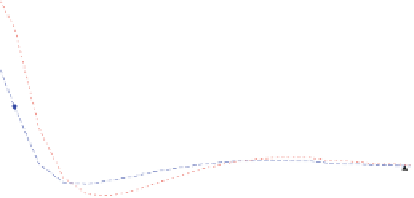















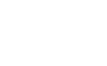

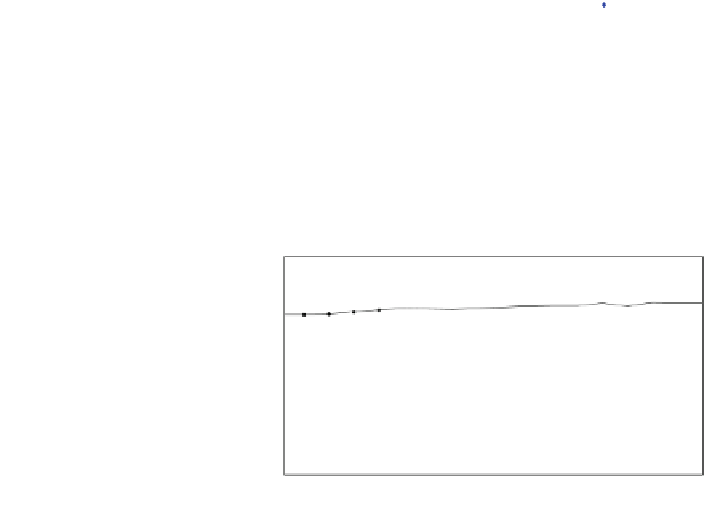










































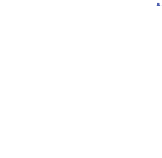





















































































































Search WWH ::

Custom Search