Information Technology Reference
In-Depth Information
and systolic blood pressure. We notice that, in addition to the clear cases, we also
have such borderline cases which only have a partial membership to this cluster.
Fuzzy cluster methods are often the corner stones when fuzzy model construction
with empirical data is carried out. Fuzzy clusters reveal us the typical cases in the
data set, and the rest of the predicted values of the models are specified according
to these objects. This approach is widely used in the regression models, and we will
consider them next with our data set.
18.3.2
Regression Models
Consider first a simple regression model in which we have
Bmi
as the independent
and
Syst
as the dependent variable. Figure 18.4 shows us that a more or less linear
correlation seems to prevail between these variables and thus a linear model is first
constructed with SPSS (Table 18.3). This model yields us the regression equation
Syst
=
3
.
454
Bmi
+
67
.
563
with the root mean square of error (RMSE) = 19
.
66 when
e
i
n
)
=(
∑
i
1
/
2
RMSE
,
i
=
1
,
2
,...,
n
in which
e
1
,
e
2
, ...,
e
n
are the error terms (residuals) and
n
is the number of cases.
A cubic curve, i.e., a third degree polynomial, would now yield an almost similar
RMSE.
Table 18.3
Regression coefficients for a linear model
Syst
vs.
Bmi
Unstardardized Coefficients Stardardized Coefficients
t
Sig.
B
Std. Error
Beta
Bmi
3.454
.435
.630
7.940 .000
(Constant) 67.563
11.308
5.975 .000
As an example of a fuzzy model, we apply a system with six initial fuzzy rules
which are actually six cluster centers of our data (Table 18.4). Hence, according to
the first center, we may generate the corresponding initial fuzzy rule.
If
Bmi
is approx. 20
.
5, then Systolic blood pressure is approx. 120.
In our model we apply the first-order Takagi-Sugeno reasoning [19] and thus
the original consequents of the rules are replaced with linear functions (Fig. 18.5).
Our RMSE is now 18.86 which is slightly better than in the foregoing traditional
models. Figure 18.6 depicts our fitting and we notice that even complicated non-
linear fittings may be obtained conveniently with fuzzy systems.
Next, we add another independent variable to our model, viz. the age of persons.
Figure 18.7 depicts our data set in a 3-D space, and our task is now to specify such
































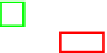
