Geology Reference
In-Depth Information
This section gave an overview of modeling results of some fairly recent AI
techniques on the topic of evaporation estimation. The analysis of this kind is one of
the few initial attempts to apply arti
cial intelligence data-based models such as
ANN, SVM, and ANFIS models in a Middle East region. The study has con
rmed
that the models LLR, SVMS, ANN, and ANFIS worked well for a hot and dry place
such as Iran. However, the fuzzy element introduced by ANFIS and the basic SVM
failed to outperform the ANN-LM in this study, so it would be interesting to
explore its ability when applied in conjunction with discrete wavelets.
7.6.2 Evaporation Modeling with Hybrid Models NNARX,
NW, W-ANFIS, and W-SVM
For the purpose of constructing a NNARX model, a multi-layer feed-forward
network type of arti
cial neural network (ANN) was built on the basis of a linear
ARX model with inputs such as that of an ARX model. The neuro-wavelet (NW)
model was constructed using a multi-layer feed-forward network type of arti
cial
neural network along discrete wavelet transfer (DWT) model. The discrete wavelet
transfer model decomposed the original evaporation data using high-pass and low-
pass
cients (D) and approximation (A) sub-
time series. In this NW model for evaporation modeling, selected sub-series of the
original data directly were used as inputs of the ANN model. The diagrammatic
filters into series, namely detailed coef
Fig. 7.34 The decomposed wavelet sub-time series components and approximation series of daily
records from the Chahnimeh reservoirs region: (a) daily air temperature; (b) daily wind velocity;
(c) daily saturation vapor pressure deficit (Ed)
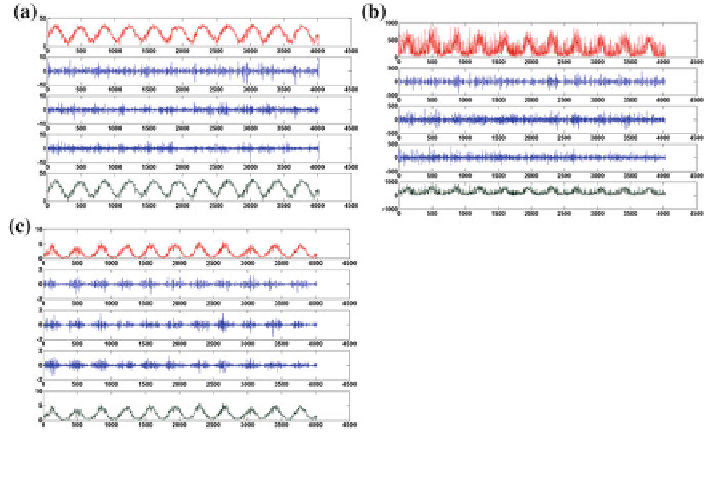