Information Technology Reference
In-Depth Information
2
2
f(e)
f(e)
1.5
1.5
1
1
0.5
0.5
e
e
0
0
−4
−2
0
2
4
−4
−2
0
2
4
(a)
(b)
2
2
f(e)
f(e)
1.5
1.5
1
1
0.5
0.5
e
e
0
0
−4
−2
0
2
4
−4
−2
0
2
4
(c)
(d)
Fig. 3.1 An error PDF (a) converging to a Dirac-
δ
by gradient ascent of the
information potential with
η
=0
.
1
; (b), (c) and (d), show the PDF at iterations
28, 30, and 31, respectively.
this case
P
e
=0. Moreover, note that computer rounding of
d
to1means,
in terms of the thresholded classifier outputs (see 2.2.1), that the two-Dirac-
δ
solution strictly implies
P
e
=0
.
5! This effect is felt for small values of
the initial
σ
,
σ
initial
, (the asymptotic
d
is 1 with five significant digits for
σ
initial
0
.
32). In short, minimization of the error entropy behaves poorly
in this case.
Example 3.1 illustrates the minimization of theoretical error entropy leading
to very different results, depending on the initial choice of parameter vectors.
In this example the initial [1 0
.
9]
T
and [1 0
.
4]
T
parameter vectors influenced
how the (
d, σ
) space was explored, in such a way that they led to radically
different MEE solutions. The basic explanation for this fact was already given
in Sect. 2.3.1: any Dirac-
δ
comb will achieve minimum entropy (be it Rényi's
quadratic or Shannon's), therefore what really matters in Example 3.1 is
achieving
σ
=0;thevalueof
d
is much less important. One may also see
both final solutions as an example of Property 1 mentioned in Sect. 2.3.4.
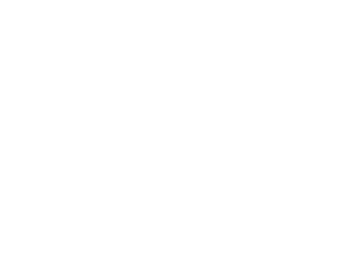
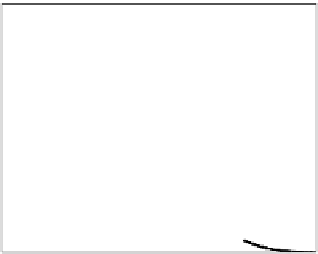


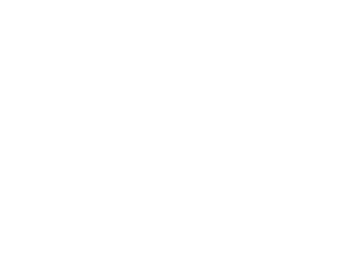
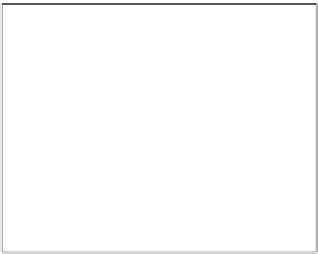














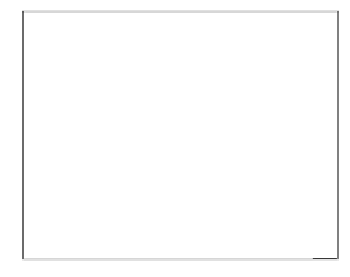








































































































