Information Technology Reference
In-Depth Information
Fig. 7.11
Recognition rate
versus subspace dimension
for different feature extraction
techniques in Indian face
database
•
Class distinctiveness with
R
ICA
is slightly better than PCA but it is best with
C
ICA
.
•
The recognition system developed with
C
ICA and
C
RSP neuron is outperforming
in all respect over others.
This makes
C
ICA
to perform very well in noisy and blurred images, which are
generally captured in real environment applications. The comparative assessment of
different feature extraction methods as a function of the dimension of compressed
subspace is presented in Fig.
7.11
. It is obtained from best performing
C
RSP classi-
fier.
C
ICA
outperforms to all other methods, it is statistically more significant when
we consider a lesser number of subspace dimensions. The relative ordering of the
subspace projection techniques depends on the number of subspace dimensions. The
performance of
C
PCA
is better in lower dimensions while
R
PCA
is better in higher
dimensions. It can be seen that the recognition rate with
C
ICA
is always higher than
any other technique.
7.6 Robustness Toward Partial Occlusion, Blurring,
and Noises
In order to examine the performance of different techniques in varying environmental
conditions, the occlusion has been simulated at random locations in few images, as
in Figs.
7.12
and
7.13
. Some of the noises and blurring has also been introduced
(electronically modified) [
14
,
34
] in other images of face data base for experiments,
as in Fig.
7.14
. In this experiment, we consider the best performing classifier of
C
RSP
neurons. Both
PCA
-based face recognizers have fairly recognized the occluded faces
shown in Fig.
7.12
where the degree of occlusion is quiet less. On increasing the
degree of occlusion (Fig.
7.13
), it is observed that the performance of both
R
PCA
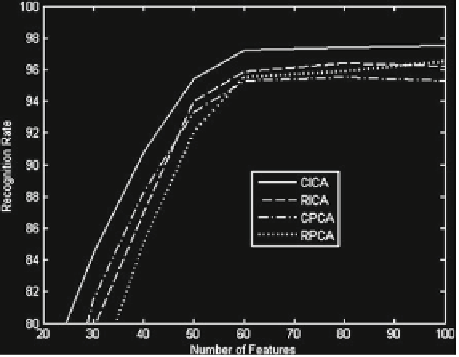
Search WWH ::

Custom Search