Information Technology Reference
In-Depth Information
(
)
=
ˆ
ˆ
ˆ
ˆ
ˆ
(
)
bbbbb
0 7 1 4 2
12 17
,
,
,
,
., ., .,., .
-
1 83
-
1 58 0 56
-
5 17
The realization h of Gregory's rule on his 155 chronic hepatitis
patients predicts that a new patient with covariate vector
t
0
will die if his
predicted probability of death
F
q
(
t
0
) is greater than
2
; that is,
logit
ˆ
()
=
q
t
12 17
.
-
1 83
.
t
-
1 58
.
t
+
0 56
.
t
-
5 17
.
t
≥
(3.2)
0
0 17
.
0 11
.
0 14
,
0 3
,
For the dichotomous problem, we use the criterion
(
)
=
Qy
,
h
1
0
if
otherwise
y
π
h
,
=
q
The apparent error is
app
= 0.136. Figure 1 shows a histogram of
B
= 400
bootstrap replications of
R
* =
R
( *, ). Recall that each
R
* was calcu-
lated using (2.1), where h
*
is the realization of Gregory's rule on the
bootstrap sample
x
1
,...,
x
n
. The bootstrap estimate of expected excess
error was
F
F
F
B
1
*
=
Â
ˆ
r
~
R
b
0 039
.
.
boot
B
b
=
1
The jackknife and cross-validation estimates were calculated to be
ˆ
ˆ
r
=
0 023
.
,
r
=
0 019
.
.
jack
cross
Adding expected excess error estimates to the apparent error gives bias-
corrected estimates of the error:
ˆ
ˆ
ˆ
q
=
0 175
.
,
q
=
0 159
.
,
q
=
0 145
.
.
boot
jack
cross
All three estimates require substantial computing time. FORTRAN
programs for performing the preceding calculations and the ones in the
following section were developed on a PDP-11/34 minicomputer. The
cross-validation and jackknife estimates were computed in 1
2
hours,
whereas the 400 bootstrap replications required just under 6 hours. Com-
puters are becoming faster and cheaper, however, and even now it is possi-
ble to compute these estimates on very complicated prediction rules, such
as Gregory's rule.
Are
B
= 400 bootstrap replications enough? Notice that
R
1
,...,
R
B
is a
random sample from a population with mean
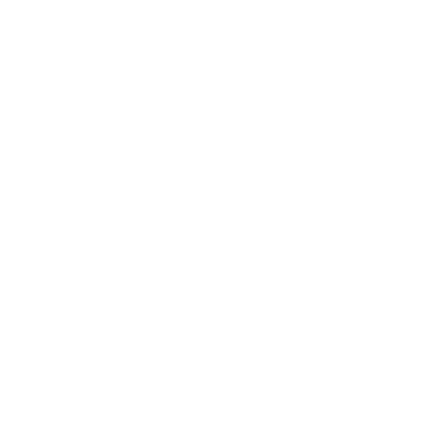

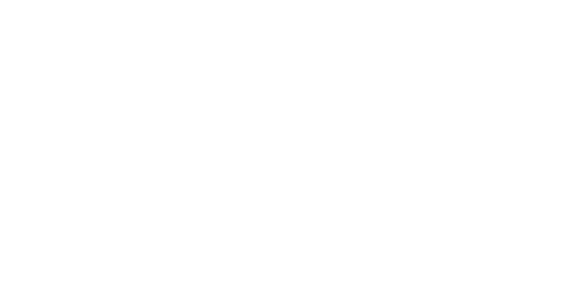



