Environmental Engineering Reference
In-Depth Information
When data uncertainty is taken into account, the
error
e
j
can be modified as
ance, where the error variance can be determined
directly from the specified coefficient of variation of the
individual measurements.
j
L
ˆ
j
U
0
if
if
if
y
≤
y
≤
y
j
11.3.2.3 Coefficient of Determination.
The
coefi-
cient of determination
,
R
2
, is the square of the Pearson's
product-moment correlation coefficient and describes
the proportion of the total variance in the observed data
that can be explained by the model. It ranges from 0.0
to 1.0, with higher values indicating better agreement,
and is given by
j
U
ˆ
ˆ
j
U
e
=
y
−
y
y
>
y
(11.22)
j
j
j
j
L
ˆ
ˆ
j
L
y
−
y
y
<
y
j
j
Therefore, when applying conventional goodness-of-
fit tests expressed in terms of the error given by Equa-
tion (11.21), uncertainty in observed data can be taken
into account by using Equation (11.22) to express the
error in each model-predicted data point (Harmel and
Smith, 2007).
2
(
∑
N
)
(
)
ˆ
ˆ
y
−
y y
−
y
j
j
j
=
1
(11.25)
R
2
=
Error Probability Distribution.
The uncertainty in an
observation can be accounted for by expressing each
observation by either a pdf or a cumulative distribution
function (cdf). A single probability distribution can be
applied to all measured data or a unique distribution
can be applied to each observation. In either case, the
error
e
j
in a goodness-of-fit statistic, defined by Equa-
tion (11.21), is replaced by
N
N
(
)
∑
∑
2
(
)
2
ˆ
ˆ
y
−
y
y
−
y
j
j
j
=1
j
=1
where
y
is the mean value of
y
predicted by the model.
The effectiveness of
R
2
is reduced when the model pre-
dictions are biased (McCuen et al., 2006). Typically,
R
2
values greater than 0.5 are considered acceptable
(Santhi et al., 2001; Van Liew et al., 2003). Although
R
2
has been widely used for model evaluation, this statistic
is oversensitive to high extreme values (outliers) and
insensitive to additive and proportional differences
between model predictions and measured data (Legates
and McCabe, 1999).
In an example application, White and Chaubey
(2005) used the
R
2
statistic to assess the performance of
the SWAT model in simulating the flow, sediment, and
nutrient yields from the Beaver Reservoir watershed
(Arkansas). Values of
R
2
ranged from 0.41 to 0.91, which
were characterized as typical of multisite, multivariable
model calibrations.
CF
(
)
j
ˆ
(11.23)
e
=
y
−
y
j
j
j
0.5
where CF
j
is a correction factor based on the probability
distribution of the observation
y
j
, and
y
j
is the model-
predicted value of
y
j
. Equation (11.23) assumes that the
probability distribution of each measured value is sym-
metric and that each measured value represents both
the mean and median value of that distribution. Under
these assumptions, the correction factor, CF
j
, is used to
adjust each deviation based on measurement uncer-
tainty. As shown in Equation (11.23), CF
j
is divided by
0.5, which is the maximum probability for one-sided
pdfs to represent the proportion of the deviation that is
accounted for by the probability distribution of each
y
j
.
The correction factor, CF
j
, can be calculated using the
relation
11.3.2.4 Model Eficiency.
Model efficiency,
or
coef-
ficient of efficiency,
,
E
, is a dimensionless standardized
measure that can be used to compare models derived
from data sets of different length and is given by (Nash
and Sutcliffe, 1970)
(
)
ˆ
ˆ
P y
< <
y
y
if
if
y
>
y
j
j
j
j
∑
∑
N
CF
=
(11.24)
(
)
2
ˆ
y
−
y
j
(
)
ˆ
ˆ
P y
< <
y
y
y
≤
y
j
j
j
j
j
j
j
=
1
E
=
1.0
−
(11.26)
N
2
(
y
−
y
)
j
where
P
(
y
) is the cumulative probability distribution
function of the observed data
y
. Application of Equa-
tion (11.24) to observed data that have normal and tri-
angular pdfs can be found in Harmel and Smith (2007).
Adjustment of model errors using Equation (11.23)
is sometimes referred to as
error-based weighting
. A
variation of the error-based weighting approach sug-
gested by Foglia et al. (2009) is to weight the model
discrepancies (
y
j
=
1
The model efficiency,
E
, is sometimes called the
Nash-Sutcliffe efficiency
(NSE) (S.K. Mishra et al., 2006;
Ritter and Muñoz-Carpena, 2006),
Nash-Sutcliffe coef-
ficient
(Wang et al., 2008),
efficiency index
(McCuen et
al., 2006), or
Nash-Sutcliffe index
(Jain and Sudheer,
2008). Equation (11.26) includes the ratio of two sums-
of-squares, where the numerator measures the variation
−
ˆ
) by the inverse of the error vari-
y
j
j
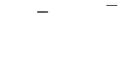



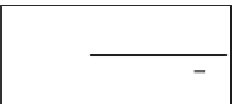



Search WWH ::

Custom Search