Environmental Engineering Reference
In-Depth Information
Residual Sum of Squares.
The residual sum of squares
is given by
The SE is sometimes used interchangeably with the
RMSE.
N
Relative Error.
The relative error is used to evaluate the
prediction accuracy of a single output variable and is
given (in percent) by
∑
y
(
)
2
RSS =
−
y
ˆ
(11.14)
j
j
j
=
1
Mean Square Error.
The mean square error is given by
y
−
y
j
j
RE =
×
100
(11.19)
y
j
N
1
∑
(
)
2
ˆ
MSE =
y
−
y
(11.15)
j
j
N p
−
and the mean relative error (MRE) is useful in measur-
ing the ability of a model to accurately simulate peak
runoff rates, and is given by
j
=
1
where
p
is the number of parameters in the model.
N
ˆ
100
y
−
y
∑
Root Mean Square Error.
The root mean square error
is an absolute error measure that quantifies the error in
terms of the units of the variable, and is given by
j
j
MRE =
(11.20)
N
y
j
j
=
1
In assessing the performance of a model in simulat-
ing observed data, it is generally advisable to use several
independent statistical measures. The set of statistical
measures typically varies with the variable of interest.
Each of the statistics mentioned above provide a differ-
ent picture of model performance, and so it is usually
preferable to use multiple statistics to assess model per-
formance. Bias shows how the model predictions
compare with observations in an overall sense, and
MAE and and RMSE show the degree of scatter or
variability between individual forecasts and observa-
tions. Biases, MAEs, and RMSEs close to zero have the
best agreement with observations.
N
1
∑
(
)
2
ˆ
(11.16)
RMSE =
y
−
y
j
j
N
j
=
1
The RMSE statistic can be considered to be most
useful, since it is directly comparable to the measured
data points. RMSE values should be assessed relative to
the uncertainty of the observations. Based on the rec-
ommendation by Singh et al. (2005), a model evaluation
statistic, named the RMSE-observations standard devi-
ation ratio (RSR), was developed. RSR standardizes
RMSE using the observations standard deviation, and
is calculated using the relation
11.3.2.2 Modified Error Statistics.
goodness-of-fit
measures typically do not account for uncertainty in
the observed data, where uncertainty in observed data
is typically characterized by the following measures:
(1) an error range; and/or (2) a probability distribution.
Modifications to the goodness-of-fit tests that take these
measures into account are described below.
∑
∑
N
(
)
2
ˆ
y
−
y
j
j
j
=
1
RSR =
(11.17)
N
(
y
−
y
)
2
j
j
=
1
where
y
is the mean of the observations. RSR varies
from the optimal value of 0, which indicates zero RMSE
or residual variation, and therefore perfect model simu-
lation, to a large positive value.
As an example application, Henriksen et al. (2003)
used the RSR statistic to measure the deviation between
predicted and observed groundwater heads.
Error Range.
The error,
e
, in observed data can be
characterized by an absolute range, such as ±
e
, or char-
acterized by a percentage error (±%) associated with
each observation. Specification of error ranges by per-
centage errors is more common. Regardless of the
method used to specify the error range, the
j
th observa-
tion,
y
j
, has an upper and lower error bound which can
be represented by
y
j
U
and
y
j
L
respectively. Most error
statistics are expressed in terms of the difference
between observations,
y
j
, and model predictions,
y
j
, such
that the error in the
j
th data point predicted by the
model is given by
Standard Error.
The standard error is given by
N
1
∑
(
)
2
SE =
y
−
y
ˆ
(11.18)
j
j
N
−
1
j
=
1
where the term
N
− 1 is sometimes replaced
N
, which
produces comparable results for large values of
N
.
−
ˆ
e
=
y
y
(11.21)
j
j
j

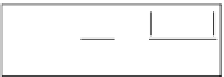
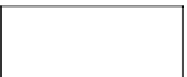

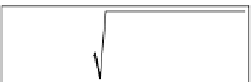

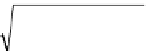
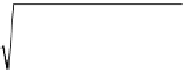




Search WWH ::

Custom Search