Environmental Engineering Reference
In-Depth Information
TABLE 10.12. Commonly Used Intrinsic Semivariograms
n
∑
λγ
(
x
−
x
)
+
α γ
=
(
x
−
x
)
,
i
=
1
,
…
,
n
j
i
j
i
Model
Expression
j
=
1
(10.197)
∑
n
λ
=
1
0
if
if
h
= 0
0
i
linear
γ
(
h
=
i
=
1
θ
+
θ
h
h
>
1
2
Power
γ
(
h
) =
θh
s
, where
θ
>
0 and 0 <
s
<
2
The solution of this system of equations gives the
station weights,
λ
i
, to be used in kriging based on the
intrinsic hypothesis. Combining Equations (10.195) and
(10.197), the variance of the estimation error can then
be estimated using the relation
0
if
h
=
0
3
3
2
h
1
2
h
Spherical
γ
( )
h
=
θ
+
θ
−
if
0
< <
h
θ
1
2
3
θ
θ
3
3
θ
+
θ
if
h
≥
θ
1
2
3
0
if
h
=
0
n
∑
ˆ
ˆ
Exponential
γ
( )
h
=
h
var(
Z Z
−
)
=
(
Z Z
−
)
2
=
λγ
(
x
−
x
)
+
α
(10.198)
θ
+
θ
1
−
exp
−
if
h
>
0
i
i
1
2
θ
i
=
1
3
0
if
h
=
0
2
The basis for estimating the kriging weights in the
intrinsic case is the semivariogram,
γ
(
h
). In practice,
γ
(
h
) is first calculated from synoptic field measurements,
where a semivariogram calculated from field data is
called the
experimental semivariogram
or
empirical
semivariogram
. The definition of the semivariogram
is given by Equation (10.186), and the empirical
semivariogram
Gaussian
γ
( )
h
=
h
θ θ
+
1
−
exp
−
if
h
>
0
1
2
θ
3
logarithmic
γ
(
h
) =
A
log(
h
), where
A
>
0
is commonly calculated using the
of the linear model
(Piegorsch and Bailer, 2005), the
nugget is
θ
1
, and the sill is
θ
1
+
θ
2
. This is a case where
the sill is never actually attained by the semivariogram
function, but instead is a true asymptote. As a conse-
quence, the range is undefined. For analysts who define
the range as the point where
γ
(
h
) attains some majority
percentage of the sill, the exponential model presents a
complication that such a range will always be a function
of
θ
3
, but never exactly equal to it. The best interpreta-
tion one can give
θ
3
under this model is that it controls
the rate at which
γ
(
h
) approaches
θ
1
+
θ
2
. In the Gauss-
ian model shown in Table 10.12, the nugget is
θ
1
, the sill
is
θ
1
+
θ
2
, and the sill is never actually attained, hence
the range is undefined. The parameter
θ
3
has the same
interpretation as the exponential model.
relation
1
∑
ˆ
γ( )
h
=
{ ( )
Z
s
−
Z
(
s
)}
2
(10.199)
i
j
2
N
h
( , )
i j G
h
∈
where
G
h
is the set of all pairs of indices whose corre-
sponding points (
s
i
,
s
j
) satisfy
s
i
−
s
j
=
h
, and
N
h
is the
number of distinct pairs of indices (points) in
G
h
. As
illustrated by Equation (10.199), the empirical semivar-
iogram is calculated for a given value of
h
by finding all
the points separated by
h
and calculating half the
average squared deviation between them.If the process
is isotropic,
h
can be replaced by the scalar distance |
h
|.
The experimental semivariogram is commonly fitted
to an analytic function. In most cases, the analytic semi-
variogram is assumed to be isotropic, and some of the
commonly used analytic semivariograms are listed in
Table 10.12. The power model includes the widely used
linear model,
γ
(
h
) =
θh
, as a special case, and the loga-
rithmic model should only be used for variables aver-
aged over finite volumes (i.e., regional variables), and
cannot be used to describe point measurements (Kitani-
dis, 1993). In the linear model shown in Table 10.12, the
nugget is
θ
1
, and the sill and range are both undefined,
since this semivariogram does not converge to an
asymptote as
h
→ ∞. The nugget under the spherical
model is
θ
1
, the sill is
θ
1
+
θ
2
, and the range is
θ
3
. In the
exponential model, also called the
exponential variant
EXAMPLE 10.32
Measurements of transmissivity in an aquifer suggest a
semivariogram of the form
γ( )
h
= 56 5
.
h
1 2
.
where
h
is in meters and
γ
(
h
) is in m
4
/d
2
. The mean
transmissivity in the region is estimated to be 2000 m
2
/d.
Estimate the transmissivity,
T
, at location
X
, based on
the measured transmissivity at four nearby stations
where the coordinates and transmissivity measurements
are given by





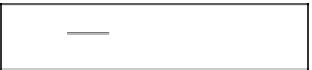








Search WWH ::

Custom Search