Environmental Engineering Reference
In-Depth Information
Several random functions are particularly useful in
characterizing the distribution of random variables. The
first is simply
+∞
∫
f x
( )
≥
0
and
f x dx
(
′
)
′ =
1
(10.1)
−∞
The cumulative distribution function (CDF), denoted
by
F
(
x
), describes the probability that the outcome of a
random process will be less than or equal to
x
and is
related to the probability density function by the
equation
g x
( ) =
x
(10.9)
In this case,
E
(
g
) =
E
(
x
) corresponds to the arithme-
tic average of the outcomes over an infinite number of
realizations. The quantity
E
(
x
) is called the
mean
of the
random variable, and is usually denoted by
μ
x
. Accord-
ing to Equation (10.8),
μ
x
for a continuous random vari-
able is defined by
x
∫
F x
( )
=
f x dx
(
′
)
′
(10.2)
−∞
which can also be written as
+∞
∫
µ
x
=
xf x dx
( )
(10.10)
dF x
dx
( )
(10.3)
−∞
f x
( )
=
A second random function that is frequently used is
In describing the probability distribution of more than
one random variable, the
joint probability density func-
tion
is used. In the case of two variables,
X
and
Y
, the
probability that
x
will be in the range [
x
,
x
+ Δ
x
] and
y
will be in the range [
y
,
y
+ Δ
y
] is approximated by
f
(
x
,
y
)Δ
x
Δ
y
, where
f
(
x
,
y
) is the joint probability density
function of
x
and
y
. other probability distributions that
are derived from the joint probability density function
are the
bivariate CDF
,
F
(
x
,
y
),
marginal probability
density functions
,
g
(
x
) and
h
(
y
), and the
conditional
probability density function
,
p
(
x
|
y
0
), are defined as
g x
( )
=
(
x
− µ
2
)
(10.11)
x
which equals the square of the deviation of a random
outcome from its mean. The expected value of this
quantity is referred to as the
variance
of the random
variable, and is usually denoted by
σ
2
. According to
Equation (10.8), the variance of a continuous random
variable is given by
+∞
∫
σ
2
=
(
x
−
µ
)
2
f x dx
( )
(10.12)
x
x
x
y
−∞
∫
−∞
∫
F x y
( ,
)
=
f x y dx dy
(
′
,
′
)
′
′
(10.4)
−∞
The square root of the variance,
σ
x
, is called the
stan-
dard deviation
of
x
and measures the average magnitude
of the deviation of the random variable from its mean.
random outcomes occur that are either less than or
greater than the mean,
μ
x
, and the symmetry of these
outcomes about
μ
x
is measured by the
skewness
or
skew-
ness coefficient,
, which is the expected value of the
function
∞
∫
g x
( )
=
f x y dy
( ,
)
(10.5)
′
′
−∞
∞
∫
h y
( )
=
f x y dx
(
′
,
)
′
(10.6)
−∞
p x y
(
|
0
)
=
f x y
( ,
)
(10.7)
0
where
p
(
x
|
y
0
) is the probability density of
x
given that
y
0
is in the range [
y
0
,
y
0
+
dy
0
]. Similar expressions can
be derived from joint probability density functions of
more than two variables.
(
x
− µ
σ
)
3
x
g x
( )
=
(10.13)
3
x
If the random outcomes are symmetrical about the
mean, the skewness is equal to zero; otherwise, a non-
symmetric distribution will have a positive or negative
skewness. There is no universal symbol that is used to
represent the skewness, but in this text, skewness
will be represented by
g
x
. For continuous random
variables,
10.2.2 Mathematical Expectation and Moments
Assuming that
x
is an outcome of the random variable
X
,
f
(
x
) is the probability density function of
X
, and
g
(
x
)
is an arbitrary function of
x
, then the expected value of
g
, represented by
E
(
g
), is defined by
1
3
+∞
∫
3
+∞
g
=
(
x
−
µ
)
f x dx
( )
(10.14)
∫
E g
( )
=
g x f x dx
( ) ( )
(10.8)
x
x
σ
−∞
x
−∞
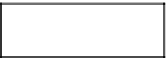
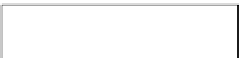
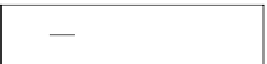


Search WWH ::

Custom Search