Information Technology Reference
In-Depth Information
(a)
(b)
Fig. 8.6.
Contrast stretched images (originals in Fig. 8.3): (a) from high-contrast address
window variant; (b) from low-contrast red ink variant of the Data Matrix codes.
(a) (b) (c)
Fig. 8.7.
Problems with adaptive thresholding (shown are a part of the original image as well
as the corresponding part of the contrast stretched image): (a) vertical dark line; (b) vertical
bright line; (c) high noise.
white version of the original Data Matrix. However, closer inspection of the contrast
stretched low-contrast images reveals some problems, as illustrated in Fig. 8.7. Most
problematic outputs are either due to printing errors (vertical dark or bright lines)
or due to noise caused by the paper of the envelope. These cases warrant further
attention.
8.4 Image Degradation
The learning of image processing tasks is based on the idea that one can use the out-
put of a simple method for unproblematic examples as desired output for a network
that is trained to produce them even if the unproblematic examples are degraded.
In the case of binarization of Data Matrix codes, a Neural Abstraction Pyramid is
trained to produce the output of the adaptive thresholding method not only for the
original images, but for degraded versions of them as well. Degradation is done by:
-
adding vertical dark and bright lines,
-
adding a smoothly varying background level,
-
lowering contrast, and
-
adding pixel noise.
These degradations are illustrated in Figure 8.8. The number of vertical lines is
uniformly drawn from the interval
[0
,
99]
. They are positioned uniformly and have




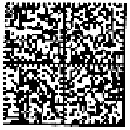



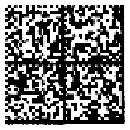
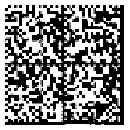


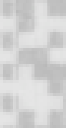

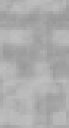

Search WWH ::

Custom Search