Environmental Engineering Reference
In-Depth Information
Table 2.1
(continued)
Method
Principle
Advantages
Disadvantages
Best suited scenario
Schematic
Inverse distance weighting (IDW)
Linear combination of
known points,
weighted inversely by
distance
Ease to use, and works well with noisy
data
Spatial arrangement of samples
does not affect weights
Moderately dense
sampling with
regard to local
variation
Splines
Fits a smooth curve to a
series of data points
Visually appealing curves or contour
lines
May mask uncertainty present
in the data
Irregularly spaced data
Radial basis functions (RBF)
Similar to the principle of
splines, except the
curve is not smooth
Required fewer samples
Required good coverage of
input space, and not suited
for extrapolation
Regions with well
distributed data
points, though
sparse
Artificial neural networks (ANN)
Learn complex patterns in
the available data
using Sigmoid
functions etc.
Ability to learn and generalize data;
works well with sparse data
distributions
Risk of poor interpolation
caused by over-learning or
under- learning
Regions ranging from
sparse irregularly
distributed data to
well distributed
data
Kriging
Similar to the principle of
IDW; however,
additionally accounts
for the spatial
arrangement
Best linear unbiased spatial predictor:
and no edge effects resulting from
trying to force a polynomial to fit
the data
Sophisticated programming
required; and problems of
non stationary in real
world data set
Well-distributed data
with no
discontinues
Topo to raster
Not specified
Based on iterative finite difference
methods. Interpolates a
hydrologically ''correct'' grid from
a set of point, line and polygon
data
Requires contour vector data as
input. Available in
ArcGIS based on
Hutchinson's ANUDEM
program
well-distributed data
points

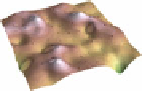
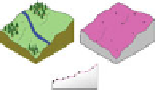
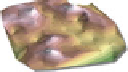
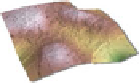
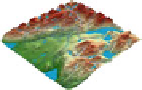





Search WWH ::

Custom Search