Information Technology Reference
In-Depth Information
1.4
50
STK−based (w=7)
Gait−based (w=7)
STK−based (w=9)
Gait−based (w=9)
STK−based (w=7)
Gait−based (w=7)
STK−based (w=9)
Gait−based (w=9)
1.2
40
1
30
0.8
0.6
20
0.4
10
0.2
0
0
0
2
4
6
8
10
0
2
4
6
8
10
Frame separation
Frame separation
(a) Processing Time
(b) Retention of Features
Fig. 20
Comparison between the STK-based and gait-based framework in tracking for the
two pedestrians sequence.
algorithm for a video sequence in which two pedestrians walk towards the camera.
Similar to the results of the slow walking sequence, the proposed algorithm pos-
sesses less feature loss and faster convergence than the STK scheme. This is due to
gradual motion of the two moving pedestrians, resulting in very slow feature loss
throughout the whole sequence.
An obvious potential weakness of the longer-term motion model is a change in
parameters as the pedestrian wearing the camera alters course. Corner turning is a
challenge to simple tracking algorithms, but a common occurrence in pedestrian se-
quences. Tracked feature points may abruptly disappear, leading to unstable motion
estimation as the reduced correspondences affect the computation of pose parame-
ters, and more errors in registration due to the appearance of additional candidates
from behind the corners. To justify the validity of the proposed gait-based method
in this case we first used the STK-based strategy to track the extracted feature points
across 100 frames (Figs. 21-23). This period allows the camera positions to be sta-
bly estimated in an entire turning period. The motion pattern of the pedestrian when
turning the corner has been accurately recovered, as seen from the regular variation
of
t
x
,
t
z
and
θ
y
of Fig. 23. However, the gait-based tracker maintains its performance
better as the frame separation increases (see Fig. 22). Without a complete (or almost
complete) walking history at the corner, one may not obtain a proper gait pattern.
With an incorrect prediction, it is unlikely that we will have correct correspondences
between frames, leading to the failure of ego-motion tracking. In our case, the suc-
cess of the gait-based algorithm can also be attributed to the slow postural changes,
i.e. the updating of the motion model is sufficiently frequent in relation to the speed
of turning the corner. However, if this were not the case, then we acknowledge that
the algorithm might not produce fast and correct motion estimates as there would
be larger errors in the extracted gait model.

































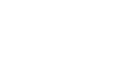
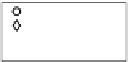





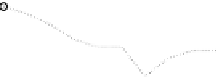






































































































Search WWH ::

Custom Search