Information Technology Reference
In-Depth Information
(a)
(b)
(c)
(d)
Fig. 2
Estimation of disparity and depth maps: (a) and (b) feature extraction, (c) disparity
map and (d) depth map
We here intend to find a strategy to achieve maximum likelihood estimation to the
flat surfaces. Let
N
independent samples be represented as
30
denoting a part of the overall image points), the probability density function
p
(
x
)
(Euclidean distance between the selected 3-D points and the fitted plane) and a
Gaussian exits as
X
=
x
1
,...,
x
N
(
N
≥
and
r
stand for a fraction of the inliers of
the estimated plane and the relationship between the samples and the inliers, re-
spectively. To obtain a maximum likelihood estimation of
N
(
x
,
θ
,
r
), where
θ
θ
and
r
, we can max-
N
imise the likelihood function
Π
i
=1
p
(
x
i
). The object function can be generalised
i
=1
as
f
(
ω
i
are weight factors and will be determined
when we carry out similarity measurements. Based on the Jensen's inequality, we
have an alternative object function as log
f
(
θ
,
r
)=
ω
i
N
(
x
i
,
θ
),where
∑
i
=1
log
ω
i
N
(
x
i
,
θ
,
r
)
q
i
,where
θ
,
r
)
≥
∑
q
i
i
=1
q
i
= 1.
q
i
is a non-negative constant that satisfies
∑
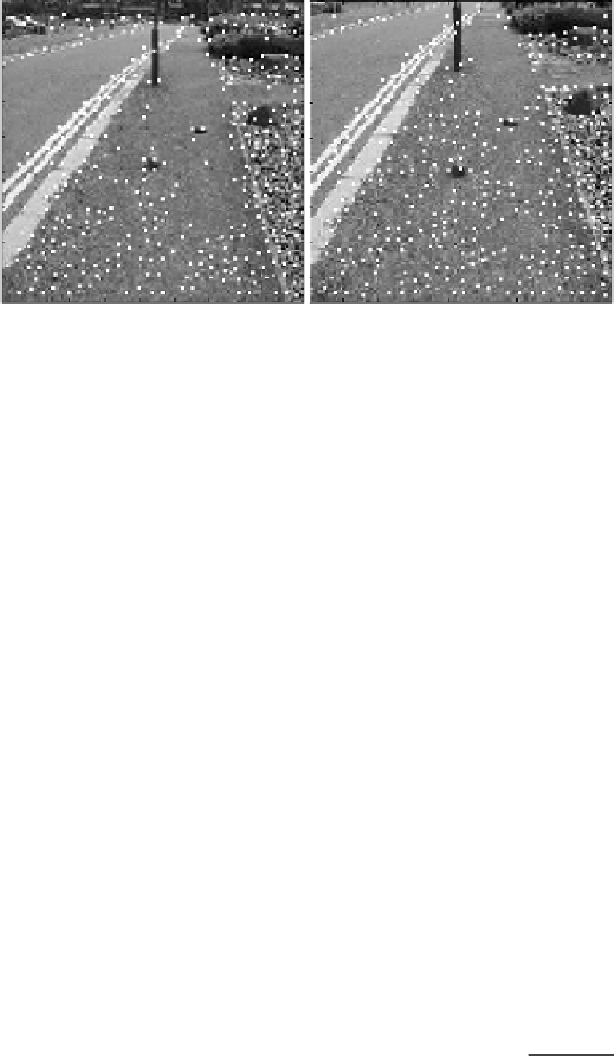
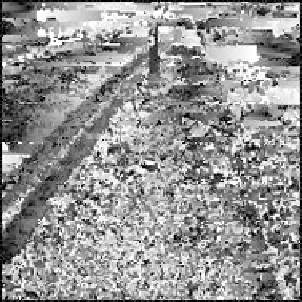
