Information Technology Reference
In-Depth Information
class 1:
+
class 2:
o
class 3:
-1.5
W
k
b
k
-1
-0.5
0
0.5
1
1.5
2
2.5
5
2
-
3
-2
-1
0
1
3.5
2
3
4
1
Fig. 3.4
Three ICA mixtures in a three-component space
highest probability was assigned to each observation vector. Figure
3.4
shows the
classification results for one of the simulated cases with 0.1 of supervision ratio
and non-parametric Mixca for updating parameters. Figure
3.4
shows three classes
with mixtures of three Laplacian pdf's. The estimated parameters (basis vectors
and bias terms) for each class are depicted on the data. In this case, the procedure
was able to find the right direction and location of the parameters. The percentage
of success in classification was 98.3.
3.4.3 Convergence Properties
The convergence properties of the procedure were empirically tested in two exper-
iments in order to evaluate the effects of the residual increment r
k
ð
i
Þ
k
¼
1...K of
Eq. (
3.13
) in different semi-supervised cases. In the first experiment, we evaluated
the log-likelihood, the SIR, and the classification accuracy achieved by the proce-
dure. A total of 1000 Monte Carlo experiments were performed using the following
parameters: (i) Number of classes in the ICA mixture K
¼
3; (ii) Number of
observation vectors per class N
¼
400 (70 % used for training); (iii) Number of
sources = 2 (Laplacians with a sharp peak at the bias and heavy tails); (iv) Super-
vision ratio (sr) = 0, 0.1, 0.2, 0.3, 0.4, 0.5, 0.6, 0.7, 1; (v) Embedded ICA algo-
rithm = Non-parametric Mixca.
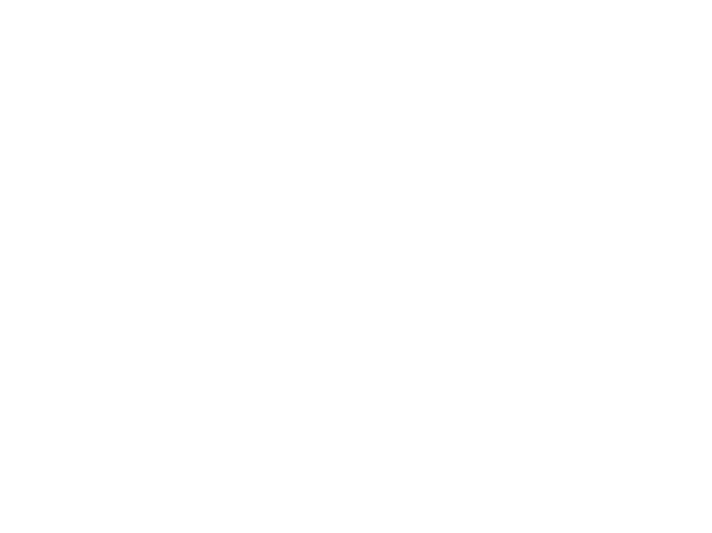










































































































































































































































































































































































































































































































































































































































































































































































































































































































































































































































































































































































































































































































































































































































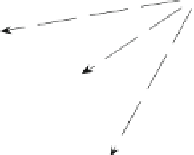






















































































































































































































































































































































































































































































































































































































































































































































































































































































































































































































































































































































































































































































































Search WWH ::

Custom Search