Information Technology Reference
In-Depth Information
Table 4.
Performance comparison of different classifiers using a separate test dataset
Accuracy
(%)
Sensitivity
(%)
Specificity
(%)
Strength
(%)
ROC
AUC
Classifier
MCC
BindN-RF
80.00
73.08
80.63
76.86
0.35
0.85
BindN
70.81
68.70
71.01
69.85
0.24
0.76
DBS-PSSM
67.91
37.48
70.72
54.10
0.05
0.55
to define DNA-binding residues. Other classifiers were constructed either using a
different dataset (including some sequences in PDC25t) or with a different definition
of DNA-binding residues.
As shown in Table 4, BindN-RF gives the best predictive performance with the pre-
diction strength at 76.86% (73.08% sensitivity and 80.63% specificity), MCC = 0.35
and ROC AUC = 0.85. Importantly, the performance measures achieved by BindN-RF
on the separate test dataset (PDC25t) are comparable with those from the fivefold cross-
validation (Table 2), suggesting that overfitting has been avoided in the construction of
the RF classifier. BindN is the second best classifier with the prediction strength at
69.85%, MCC = 0.24 and ROC AUC = 0.76. However, the ANN predictor trained with
PSSM and sequence information (DBS-PSSM) shows very poor performance on the
PDC25t dataset with only 54.10% prediction strength, MCC = 0.05 and ROC AUC =
0.55. The unexpected result for DBS-PSSM might be owing to poor generalization of
the representative DNA-binding residues in the relatively small training dataset.
1
0.8
0.6
0.4
BindN-RF
BindN
DBS-PSSM
0.2
0
0
0.2
0.4
0.6
0.8
1
False positive rate
Fig. 3.
ROC curves of three different classifiers (BindN-RF, BindN and DBS-PSSM) for se-
quence-based prediction of DNA-binding residues. The performance comparison is based on
the PDC25t test dataset.

















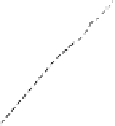






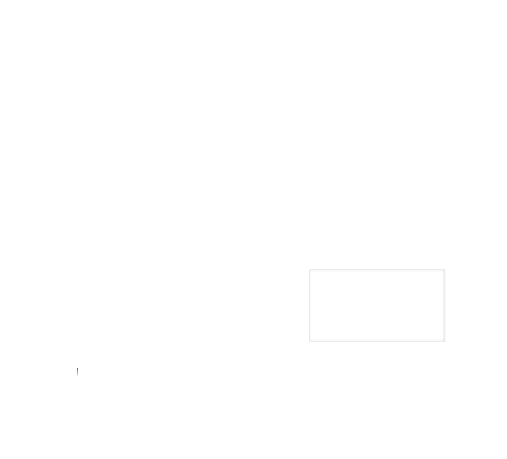




























































































Search WWH ::

Custom Search