Information Technology Reference
In-Depth Information
Fig. 7.
Pareto indices for several games and NYSE
Ta b l e 2 .
Skewness
method
ExAG
AG
MG
NYSE
average
0.098
0.39
-0.32
3.725
stdev.
1.82
1.03
1.86
—
95% int. [-0.58,0.77] [0.007,0.77] [-1.02,0.37]
—
Ta b l e 3 .
Kurtosis
method
ExAG
AG
MG
NYSE
average
42.3
72.9
148
18.92
stdev.
61.6
96.3
231
—
95% int. [21.3,67.3] [36.9,109] [62.2,235]
—
For the fifth issue, we present our results in Figure 8. The volatility is defined as the
standard deviation of the number of excess demand. The figure shows that the volatility
of AG is lower than other games for every memory size. This means the memory size
does not have a great impact on the price formation in AG.
For the sixth issue, the autocorrelation function
C
(
τ
)
is defined as
C
(
τ
)=
A
(
t
)
A
(
t
+
τ
)
,
A
(
t
)
2
where
τ
is a time lag. The value of
C
(
τ
)
becomes 1 (respectively, -1) if there is a
positive (respectively, negative) correlation between
A
(
t
)
and
A
(
t
+
τ
)
.Asshownin
Figure 9, only MG has the alternating, strong positive/negative correlation for every
time lag. Other games, AG and ExAG, have weak correlations which reduce as the time
lag grows. The practical data, NYSE, has a negative correlation only when the time lag
is
τ
=1
. Since the excess demand in NYSE is unknown, we assume the number of

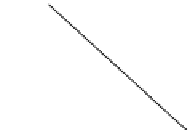


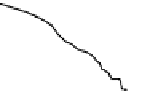

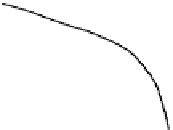
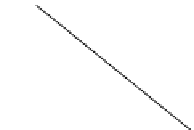





































































































































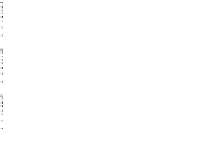




