Environmental Engineering Reference
In-Depth Information
(analogous to processes of evolutionary natural selec-
tion), and these allow agents to evaluate and select
solutions from a suite of options through time (Miller
and Page, 2007). The rise of agent-based approaches has
accompanied the growing acceptance of complexity as
a perspective that shifts understanding about economic
systems, for example, away from deterministic and pre-
dictable system behaviour towards non-equilibrium and
adaptive behaviour (for example, Arthurs, 1999). Agent-
based models (ABMs) can represent multiple interacting
social norms and behaviours (for example, satisficing,
error-prone, self-interested, altruistic) and be used to
'grow' economies and societies in a computer for analysis
in real time (Epstein and Axtell, 1996; Epstein, 2006;
Railsback and Grimm, 2012). Consequently, advocates of
ABMs have suggested they offer a means to overcome the
deficiencies of neoclassical economics in economic mod-
elling (Farmer and Foley, 2009), to anticipate and forecast
health pandemics (Epstein, 2009), for modelling land-use
and land-cover change (Parker
et al
., 2003; Matthews
et al
., 2007), representing the impact of human activ-
ity on landscape evolution (Wainwright and Millington,
2010) and many other applications.
The seminal example of agent-based modelling to
explore hypothetical societies is the Sugarscape model
(Epstein and Axtell, 1996). Sugarscape is a simplified
landscape laid out on a grid, on which a food resource
(sugar) is distributed in some initial, specified pattern.
The landscape is populated by a number of agents who
can move around the landscape according to specified
rules in order to harvest and consume the sugar. Once
harvested, the sugar is able to grow back according to
a simple set of rules. The agents are randomly assigned
a metabolism - how fast they consume the sugar they
collect, so that if their sugar resource falls to zero, they
die off. They are also assigned a distance of vision
producing differential planning of their movements,
and are randomly distributed in space at the start of
a simulation. Agents can collect more sugar than they
need to survive and thus accumulate 'wealth'. With
these simple rules, complex system behaviour emerges.
Landscapes are found to converge to specific numbers
of individuals for given sets of conditions. In other
words, a carrying capacity (see Chapter 12) emerges
from the agents' behaviour (Figure 18.3a). Furthermore,
this carrying capacity is a function of characteristics
of the agents, so that if their metabolisms and depths
of vision become dynamic properties, the carrying
capacity too would be variable. This result overcomes
a major limitation of the carrying-capacity approach to
human populations, in that the path of human history
has been one of adaptation, allowing (environmentally
imposed) carrying capacities to be overcome through
better technology, for example. It is impossible to include
500
450
400
350
300
<m>
=
1
250
<m>
=
2
<m>
=
3
200
0
2
4
Mean agent vision (number of gridcells)
(a)
6
8
10
Figure 18.3
Examples of results from the Sugarscape model of Epstein and Axtell (1996). (a) Emergent carrying capacity in numbers
of agents of a 50
×
50 cell landscape (their Figure II-5); (b) emergence of unequal distributions of wealth (measured in units of sugar)
for similar simple rules to the results shown in (a) (their animation II-3); and (c) behaviour comparing the effect of no trade in spice,
leading to extinction, with the existence of trade leading ultimately to the development of 'long wave' population oscillations (their
animation VI-2 and -3).
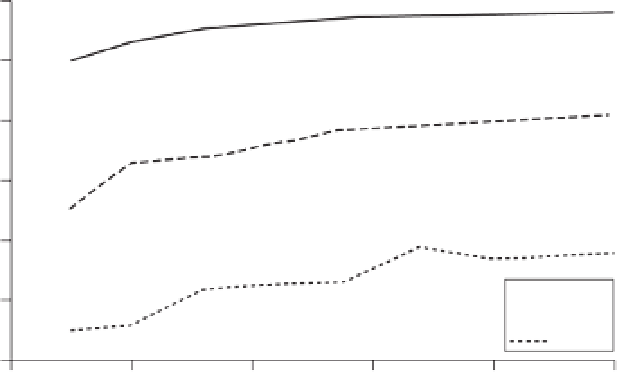



Search WWH ::

Custom Search