Environmental Engineering Reference
In-Depth Information
The DBM approach to modelling is widely applicable.
It has been applied successfully to the characterization of
numerous environmental systems including: the devel-
opment of the ADZ model for pollution transport and
dispersion in rivers (e.g. Wallis
et al
., 1989; Young, 1992,
2004); rainfall-flow modelling (Young and Beven, 1994;
Young, 2001b and the references therein); adaptive flood
forecasting and warning (Lees
et al
., 1994; Young, 2002,
2010; Romanowicz
et al
., 2006); the modelling of eco-
logical and biological systems (Young, 2000; Jarvis
et al
.,
1999); and volcanic data analysis (Young, 2006). Other
applications, in which the DBM models are subsequently
utilized for control system design, include: the modelling
and control of climate in glasshouses (e.g. Lees
et al
.,
1996), forced ventilation in agricultural buildings (e.g.
Price
et al
., 1999; Young
et al
., 2000), interurban road-
traffic systems (Taylor
et al
., 1998) and climate modelling
for emission control design (Jarvis
et al
., 2008, 2009). They
have also been applied in the context of macroeconomic
modelling (e.g. Young and Pedregal, 1999).
time series of the kind encountered in many areas of envi-
ronmental science (see Young, 1999b). For example, they
have been applied to problems such as the analysis and
forecasting of trends and seasonality in climate data (e.g.
Young
et al
., 1991); the analysis of palaeoclimatic data
(Young and Pedregal, 1998) and the analysis of plant-
physiology data (Price
et al
., 2001). The same optimized
fixed interval smoothing (FIS) algorithms used for TVP
estimation are also exploited for state-dependent param-
eter (SDP) estimation and the modelling of nonlinear
stochastic systems (see Young, 1978, 1984, 1993a, 1998,
2000, 2001a; Young and Beven, 1994).
The RIV identification and estimation algorithms can
be used for other environmental purposes. For example,
as discussed in Sections 7.7 and 7.8, they can provide
a rigorous approach to the evaluation and emulation of
large, dynamic simulation models (e.g. Young
et al
., 1996;
Young and Ratto, 2008, 2011), where 'dominant mode
analysis' provides a means of simplifying the models.
Such reduced order emulation models can then provide
a better understanding of the most important mecha-
nisms within the model; or they can provide dominant
mode models that can be used for control and opera-
tional management system design, adaptive forecasting
and data-assimilation purposes (see the references cited
in the previous section).
7.5 The statistical tools of DBM
modelling
Data-based mechanistic modelling is based on the statis-
tical estimation of dynamic models from time series data
and involves the use of powerful computer-based meth-
ods of time series analysis, most of which are available in
the CAPTAIN toolbox, developed by the authors and their
colleagues at Lancaster for use within the Matlab soft-
ware environment (see www.es.lancs.ac.uk/cres/captain/,
accessed 3 April 2012). However, the main purpose of
the present chapter is to demonstrate how these sta-
tistical tools can be used for modelling environmental
systems and not to describe the tools themselves. It will
suffice, therefore, briefly to review the various methods
of time-series analysis that are available in CAPTAIN
and assume that the interested reader will consult these
cited publications, which describe the various methods in
considerable detail.
The statistical and other tools that underpin DBM mod-
elling are dominated by powerful methods of time-series
analysis, including recursive algorithms for filtering and
smoothing. These include: optimal Refined Instrumental
Variable (RIV) methods of identifying and estimating dis-
crete and continuous-time TF models (e.g. Young, 1984,
2008), as used in the later practical examples of Sections
7.6 to 7.8; Time-Variable Parameter (TVP) estimation and
its use in the modelling and forecasting of nonstationary
7.6 Practical example
A typical practical example of DBM modelling will be con-
sidered here. It is concerned with the analysis of rainfall,
flow and temperature data from the ephemeral Canning
River in western Australia which stops flowing over sum-
mer, as shown in Figure 7.1. Although these data, which
are sampled at a sampling interval
t of one day, have
been analysed before (Ye
et al
., 1997; Young
et al
., 1997;
Young, 2004), the resulting models were identified and
estimated in discrete-time terms. Here, we consider the
alternative continuous-time approach and the advantages
associated with the resulting differential equation model.
The data plotted in Figure 7.1 illustrate well the non-
linear behaviour of the Canning River catchment. The
flow series, plotted in the top panel, reveal that there is no
flow at all in the stream during the hot summer months,
despite the fact that there are several rainfall episodes,
one of them very large. The reason for this pattern lies in
the nature of the west Australian climate and its effect on
the river catchment. During the summer months the high
temperatures and evapotranspiration completely dry the
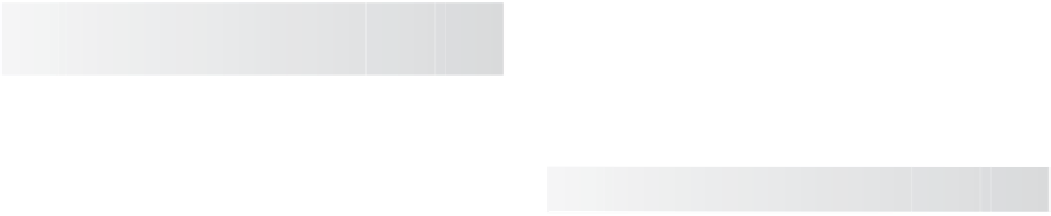



Search WWH ::

Custom Search