Information Technology Reference
In-Depth Information
Comparison of error rate in generalization
50
40
AdaBoost M1
BrownBoost
AdaBoostHyb
30
20
10
0
Databases
Fig. 3.1.
Rate of error of generalization
According to this results, we use test-student and we find a significant p-value
0.0034. We have also a average gain of 2.8 compared to AdaBoost.
Even, if we compare the proposed algorithm with BrownBoost, we remark
that for 11 databases out of 15 the proposed algorithm shows an error rate lower
or equal to BrownBoost. Using test of student, we find a p-value not significant,
But a average gain of 2.5 compared to AdaBoost.
This gain shows that by exploiting hypotheses generated with the former it-
erations to correct the weights of the examples, it is possible to improve the
performance of the Boosting. This can be explained by the calculation of the
precision of the error analysis
(
t
) and consequently the calculation of the coef-
ficient of the classifier
α
(
t
) as well as the richness of the space of the hypotheses
to each iteration since it acts on the whole of the hypotheses generated by the
preceding iterations and the current iteration.
3.4.2
Comparison of Recall
The encouraging results, found previously, enable us to proceed further within
the study of this new approach. Indeed, in this part we try to find out the
impact of the approach on the recall, since our approach does not really improve
Boosting if it acts negatively on the recall.
Graphic 2 indicates the recall for the algorithms AdaBoost M1, Brownboost
and the proposed one. We remark that the proposed algorithm has the best
recall overall the 14 for 15 studied databases. This result confirms the preceding
ones. We remark also that it increases the recall of the databases having less
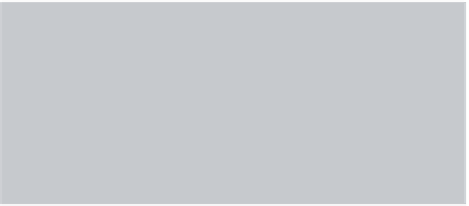
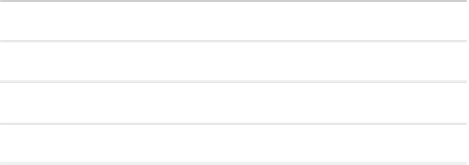






















































































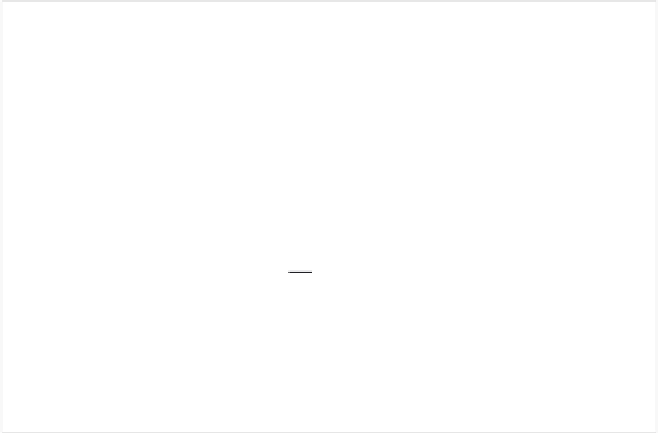


