Biomedical Engineering Reference
In-Depth Information
Fig. 5
Mean standard
deviation of the geometrical
deviation after the RPS
plotted against the varying
number of points [
18
]
1.4
RPS
Best Fit
1.2
1.0
0.8
0.6
0.4
0.2
0
6
7
11
14
16
17
21
Number of points [-]
Furthermore, the automatic, reliable and reproducible execution of this superposition
method is a great advantage over the RPS.
4.3 Agglomerative Clustering
4.3.1 Method
The universal geometry is a geometry whichmaps all underlying original geometries.
For the determination of this universal geometry, the original geometries should
be reproducibly combined. After a comparison of different possible combination
methods, agglomerative clusteringwas identified as the best method for the derivation
of the universal acetabulum geometry.
Agglomerative clustering is subsumed under the so-called data mining method.
Datamining is an approach to finding and describing existing but non-obvious knowl-
edge in collected data. For this purpose data will be analyzed and screened as to
conspicuousness, commonalities or generally to particularities [
21
,
22
]. With means
of agglomerative clustering similarities can thus be analyzed and different groups or
outliers can be identified. Individual elements will be joined in groups due to a certain
distance measure which is used as comparison criterion. The groups will be desig-
nated as cluster. The comparison criterion is defined depending on the application
[
23
,
24
].
4.3.2 Adaption of the Method
The adaption of agglomerative clustering to the derivation of the universal acetabu-
lum geometry is illustrated in Fig.
6
. Here it should be noted, that the clustering is
realized only considering the geometrical features. Characteristics like body weight
or breed are not taken into account. The whole procedure is developed on the basis
of Geomagic Qualify.
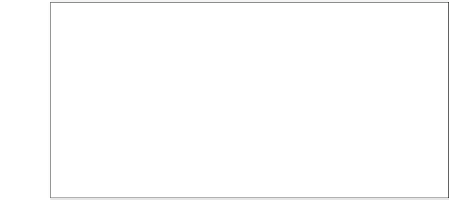









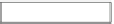



























