Information Technology Reference
In-Depth Information
−
0.04
−
0.02
0.02
0.04
1sd
11
0.06
0.08
0.1
0.12
Figure 2.7
Calibrating a biplot axis. Shown are the sample point
11
and axis
y
for the
data in Table 2.1. The origin is indicated by the green circle.
Using the above code with arguments
lambda = 3
and
shift = 0.1
results in the
much better scaling shown in Figure 2.8. Although precisely the same y-value for
11
is
read from the biplot axes in Figures 2.7 and 2.8, it is much easier to see in Figure 2.8.
Note that the approximated y-value for
11
is very close to the actual value of 0.0490.
Now consider normalizing the data matrix to unit variances and zero means: let
(
V
)
2
=
U
V
≈
(
V
2
)
2
1
/
2
X
Norm
:24
×
4
U
)
2
24
2
(
=
(
U
1
/
2
.
(2.14)
)
2
×
×
4
24
×
2
2
×
4
The right-hand side of (2.14) points to another form of scaling which we shall call
sigma scaling
. Sigma scaling refers to how the diagonal matrix
is divided between
the coordinates of the row points of
X
and the coordinates for plotting its columns.
We illustrate the calibration procedure by displaying
11
and axis
y
calibrated in
the original units although it is now not
X
that is approximated but the matrix
X
Norm
.
We first plot
(
U
)
2
:24
×
2versus
V
2
:4
×
2 in Figure 2.9 and then
(
U
/
1
2
)
2
:24
×
2
1
/
2
versus
2 in Figure 2.10. Due to all variables in
X
Norm
having zero means
the origin in both Figures 2.9 and 2.10 approximates the mean. The unscaled mean
for variable
y
is 0.0347. The y-value of
11
in
X
Norm
is 0.4676. It is this value that
(
V
)
2
:4
×






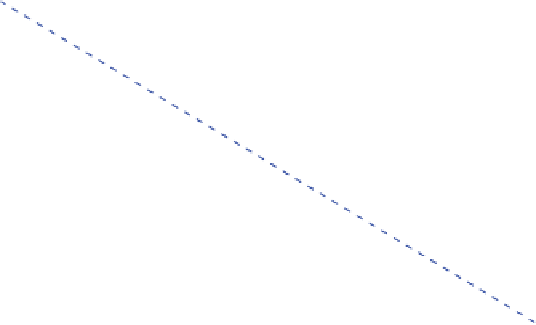
