Biology Reference
In-Depth Information
regions. However, the resulting gene regulatory networks
(GRNs) are often too complex and require filtering and
partition steps before the model can be constructed.
Largely, models in the stem cell field are focused on
a single kernel and involve few transcriptional regulators
[16
successfully modeled by starting from SINs, such as
Boolean networks. Critical network domains and kernels,
crystallized from these preliminary studies, may be
analyzed in detail, using steady-state or dynamic BRNs, as
long as the required biological knowledge is available for
the interacting genes.
22]
.
Quantitative dynamical models are biological hypoth-
eses expressed in a formal way. Quantitative models
provide room for better capturing diversity and complexity
of biological systems, but require formalization and/or
quantification of biological data. Current quantitative
predictions still require experimental validation, since the
models contain many unknown or ambiguous parameters.
Roughly, all quantitative models are focused either on
biochemical (BRN
e
Deterministic and Stochastic View
of Self-Renewal and Differentiation
One of the general key features shared by both embryonic
and adult stem cell types
[32]
is their ability to self-renew.
It is believed that self-renewal and pluripotency depend on
a relatively small set of gene network circuits (kernels)
connecting few transcriptional regulators. It is assumed that
these core transcriptional circuits occupy the top position in
the regulatory hierarchy within a stem cell of any kind and
regulate expression of thousands genes, which actually
define the self-renewal state
[5,33,34]
. In the case of
embryonic stem cells (ESCs), the regulatory kernel
involves the transcription factors Nanog, Oct4 and Sox2
[35,36]
. In the case of adult bone marrow hematopoietic
stem cells (HSCs), the potential candidate regulators
include Fli1, Scl, Gata2 and few other transcription factors
[37]
.
Figure 22.1
shows a suggested design for the two core
stem cell gene regulatory networks from vertebrates and
a retina determination network from flies
[38
biochemical reaction networks) or
statistical interactions observed between genes in gene
networks (SIN
e
statistical influence networks)
[23]
.
Typically, BRN-based models describe gene product
dynamics and rely on systems of ordinary differential
equations (ODE) or stochastic differentiation equations
(SDE)
[21,24]
, where the reaction constants are often
represented by unknown parameters. Certain BRN-based
models are limited by consideration of the reaction's steady
states (or gene response potentials) and rely on systems of
logistic functions describing, for instance, fractional
occupancy of binding sites for transcription factors (BSTF)
in the gene control regions
[25
e
27]
. Since BRN-based
models attempt to capture many biological details, they are
better to handle smaller gene networks including typically
about a dozen of genes.
A second broad class of models, based on SIN, requires
no specific knowledge regarding the already known biology
and may rely on genome-wide readings of gene expression
patterns, the results of statistical analysis of gene associa-
tions in large databases, or both. SIN-based models include
random and probabilistic Boolean models (RBN and PBN
[24]
). In the late 1960s, Kauffman
[28]
first proposed the
use of random Boolean networks to model gene regulatory
networks. More recently, probabilistic Boolean networks
(PBNs) have been developed as the stochastic extension of
classic Boolean networks
[29,30]
. In a PBN, each node
could potentially have more than one possible Boolean
function to be randomly selected at each time step, and the
resultant output of each function carries a probability.
Subsequently, the long-run steady-state behavior can be
studied in the context of Monte Carlo Markov chains. It is
assumed that concentration levels in GRNs can be
approximated by the Hill function, which in extreme cases
(high cooperativity) approaches a step function
[31]
,
therefore the Boolean formalism can capture many
dynamic properties of GRNs.
As it appears today, exploration of a complex system,
such as the self-renewal gene network in embryonic stem
cells, with largely unknown gene interactions, may be
e
40]
; all three
e
FIGURE 22.1
Functional redundancy in the core regulatory
networks. (A) Shows a minimal gene network for mouse embryonic stem
cell. (B) Retina determination core network from flies. A network with
identical elements: Six1 (So)
e
Eya2 (Eya)
e
Dach2 (Dac) is involved in the
determination of muscle cells in vertebrates. (C) The suggested minimal
core network for hematopoietic stem cells. All three networks share the
same network motif, a fully connected triad. Such a design can provide
substantial functional redundancy to the master regulator networks in
general. While the actual gene regulatory networks underlying pluri-
potency or hematopoiesis are much larger, the core elements appear to
represent central, relatively independent kernels.
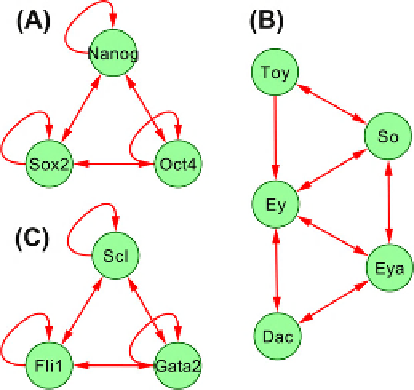


