Environmental Engineering Reference
In-Depth Information
Table 1.15
Types and parameters for the identified distributions of (Y
1
, Y
2
, Y
3
)
Distribution parameters
Random variable
Soil parameter
Distribution type
a
X
b
X
a
Y
b
Y
Y
1
LI
SU
1.491
−
1.114
0.658
0.328
Y
2
SB
2.506
2.938
16.177
ln(
σ
p
/
P
a
)
−
3.372
Y
3
ln(
S
t
)
SU
3.046
2.270
−
2.965
−
0.285
solution based on 1000 data points. The differences arise from statistical uncertainty. The
fitted distributions are shown in
Figure 1.26
—the fits are fairly satisfactory, albeit the
identification is not perfect (e.g., Y
2
is incorrectly identified as Johnson SB). For Y
1
and Y
3
,
the identified type is SU. Again, these observations can be fully explained using statistical
uncer taint y.
The rule of the game in this section is to assume that the exact solution in the form of
Table 1.14
is not available. Only the numerical values of the data points are made available
to the engineer, say in the form of an EXCEL spreadsheet containing 1000 rows and three
columns. Following the spirit of this game, the following equation can be used to transform
2
Y
−
++
−
b
Y
b
i
Y
i
i
Y
i
X
=+×
ba
ln
1
(1.102)
i
X
i
X
i
a
a
Y
i
Y
i
where (
a
X
,
b
X
,
a
Y
,
b
Y
) can be found in
Table 1.15
.
For Y
2
, the identified type is SB. Hence,
1.95
):
−
( )
−−
Y
ba
ba
2
Y
2
Y
2
X
=
ba
+
×
ln
(1.103)
(
)
2
X
2
X
2
1
Y
2
Y
2
Y
2
to standard normal. The
p
-values based on the standard normal K-S test are shown in the
figure. The large
p
-values suggest that the standard normal distribution cannot be rejected
for the converted (X
1
, X
2
, X
3
) data. That is to say, the fitted Johnson distributions cannot be
rejected for the simulated (Y
1
, Y
2
, Y
3
) data.
1.6.4 estimation of the correlation matrix C
X
2
, X
3
) data do not exhibit any nonlinear trend. The line test discussed in Section 1.4.3
line test is shown in
Figure 1.29
,
suggesting that the multivariate normal hypothesis is
suitable for the converted (X
1
, X
2
, X
3
) data. The correlation matrix
C
can be estimated
using
Equation 1.57
,
because we have full multivariate data in this simulated example.
The estimated
C
is

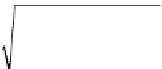














Search WWH ::

Custom Search