Environmental Engineering Reference
In-Depth Information
{
}
(
)
where
λ
is the mean value of the variable ln(
s
i
), and ξ
=
ln 1
+
δ
2
is
=
ln
µ
/1
+
δ
2
ai
i
ai
i
ai
the standard deviation of the variable ln(
s
i
).
Let
s
jl
and
s
ju
denote the lower and upper bound values of observed settlement for the
j
th
range observation, respectively. For a range observation, the observed data can be denoted
as
d
j
= {
s
jl
,
s
ju
}. Based on the lognormal distribution assumption, the chance to observe such
a range observation can be written as
ln
s
−
λ
ln
s
−
λ
−
ju
j
jl
j
P
(|)
d
θ
=
P s
(
≤
s
≤
s
|)
θ
=
Φ
Φ
(4.41)
j
jl
j
ju
ξ
ξ
j
j
Note that the database with range observations analyzed herein may be considered as a
generalization of the database with only censored data analyzed previously. For censored
data, only the lower bound or the upper bound is known; for a range observation, both the
lower and the upper bounds are known. For the database with
m
fixed-value observations
and
n
range observations, the likelihood function can be obtained by multiplying the chance
for each observation together (regardless of whether an observation is a fixed-value obser-
vation or a range observation), as long as these observations are statistically independent.
Note the chance for a fixed-value observation and a range observation can be calculated
with
Equations 4.40
and
4.41
,
respectively. Therefore, the log-likelihood function of θ can
then be written as follows:
m
2
n
‡
Ž
ln
s
−
λ
ln
s
−
λ
(
)
−
‰
‰
1
2
ln
s
−
λ
‰
‰
∑
∑
ju
j
jl
j
L
(
θ
|d
=
)
2
π ξ
s
ii
i
i
+
ln
Φ
Φ
−
ln
−
ξ
ξ
ξ
i
j
j
i
=
1
j
=
1
(4.42)
The spreadsheet template for maximizing likelihood in
Equation 4.42
is shown in
Figure
To determine the liquefaction-induced settlement in a future case,
Equations 4.34
and
4.35 are first used to compute μ
p
and σ
p
, respectively. With the knowledge of bias factor,
{μ
α
*, σ
α
*} = {1.045, 0.318}, we can compute the mean and standard deviation of the actual
settlement using
Equations 4.37
and
4.38.
4.6 SuMMarY anD ConCluSIonS
We illustrated the effectiveness of the maximum likelihood principle for calibrating proba-
bilistic models for liquefaction hazard evaluation using observed field performance data. In
this chapter, we show how the likelihood function can be constructed for different types of
data with an emphasis on applications in liquefaction evaluation. For most of the examples
explained in this chapter, the likelihood functions are well behaved and the maximum like-
lihood point can be found through a convenient use of optimization tools such as Excel
Solver. A detailed formulation of the model calibration with illustrated examples presented
in this chapter clearly show the development of these probabilistic models for evaluating
liquefaction hazards, and how they may be used in future events.
When developing models for liquefaction probability, it is generally understood that exist-
ing censored databases used for model calibration are subjected to sampling bias. Although
the weighted likelihood method can be used to adjust the effect of sampling bias, the exact
value of sampling bias is usually hard to assess due to the difficulties encountered when

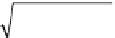



Search WWH ::

Custom Search