Geoscience Reference
In-Depth Information
Such independent validation is a powerful tool to show, for example,
consistency between air temperature, precipitation, and snow height or snow water
equivalent. Discrepancies can be, for example, well associated either to problems in
air temperature or precipitation measurements. Similar validation approaches were
also applied to climate reanalysis data.
1.2.3.
Methods for climate data homogenization
INSPECT DATA
AND METADATA
- Identify potential problems
-
Make sure data is QC'd and
free of major errors
BUILD REFERENCE
TIME SERIES
From Neighboring Stations,
being careful with:
DIRECT
HOMOGENIZATION
When sufficient parallel
measurements or experimental
data are available and reliable
adjustments can be made
-Network density
-Network-wide problems
-Meteorological element
-Time scale
-Climatic type
- Inhomogeneities in neighbors
From Independent data
(e.g. satellite)
TEST STATION DATA
IF REFERENCE T.S.
ARE NOT AVAILABLE
TEST DIFFERENCE
OR RATIO TIME
SERIES
IDENTIFY BREAKPOINTS
Plot data
Plot diference or ratio t.s.
Use statistical tests
Use metadata
ADJUST DATA
Correct Gradual Trends
Correct Jumps
ALWAYS PRESERVE
THE ORIGINAL
DATA AND RECORDS
OF ADJUSTMENTS
EVALUATE
ADJUSTMENT RESULTS
Figure 1.9.
Schematic representation of homogenization procedures for monthly
to annual climate records (from [AGU 03])
Whereas DQC is a well-established part of the standard workflow for the
database of NHMs, data homogenization still has a strong link to climate research
and not to standard procedures. This originates, in part, from the fact that powerful
homogenization methods were only developed during the last approximately 15
years. Today there exists various types of homogenization tools for climate data
involving different homogenization philosophies and with different strengths and
weaknesses regarding climate element, geographical region, or temporal resolution
of the dataset ([AGU 03]). It can be concluded from literature (e.g. [AUE 07],
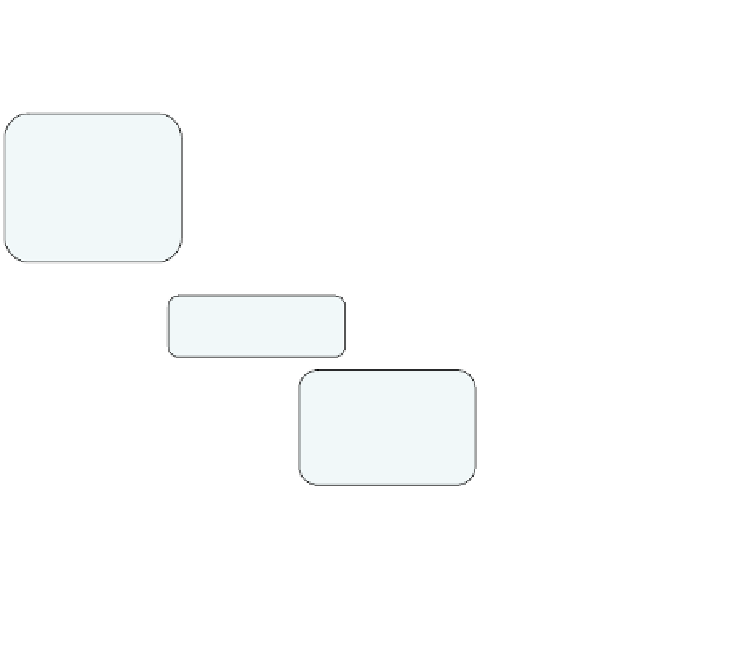

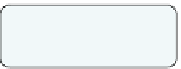
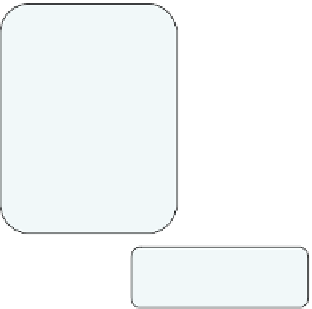






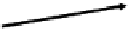





