Geoscience Reference
In-Depth Information
Fig. 12.1 Bias reduction in
grouped jackknife; the
expectation
E
n
as a function
of
n
(Source: Efron
1982
,
Fig. 2.1)
12.1.1 Abitibi Volcanogenic Massive Sulphides Example
Can the bias reducing capability of the jackknife be used in spatial situations in
which there is complete information on a set of explanatory variables but incom-
plete information on the dependent variable or probability of occurrence that is to
be estimated? The following example produced results that are encouraging but
further research would have to be undertaken to establish more widespread appli-
cability. Other publications reporting tentative results along the same lines include
Chung and Agterberg (
1980
) and Fabbri and Chung (
2008
).
Agterberg (
1973
) investigated the possibility of using the jackknife for elimi-
nating bias in mineral potential mapping where bias is introduced by likely exis-
tence of undiscovered mineral deposits in a study area. Large deposits for several
metals in the Abitibi area on the Canadian Shield were taken for example. Appli-
cations of stepwise regression to relate occurrences of large copper deposits to
regional geological and geophysical variables were previously discussed in Sect.
contained in (40
40 km) cells had been corrected for bias because of undiscovered
copper deposits by assuming that all large deposits near the surface of bedrock had
been discovered in a control area constructed around the relatively well explored
eliminate this type of bias instead of making the assumption involving a
control area.
In the application to copper deposits in the Abitibi area (Agterberg
1973
)a
grouped jackknife was used. In general, a set of
N
data can be randomly divided
it
k
subgroups each consisting of
n
data so that
k
N
. Suppose a statistic
Y
is
estimated from all data yielding
Y
all
; and also
k
times from sets of (
N
-
n
) data, which
gives
k
estimates
n
¼
Y
j
(
j
Y
j
¼
Y
all
¼
1,
...
,
k
). The so-called pseudo-values
k
Þ Y
j
are averaged to obtain the jackknife estimate
Y
and its variance
ð
k
1
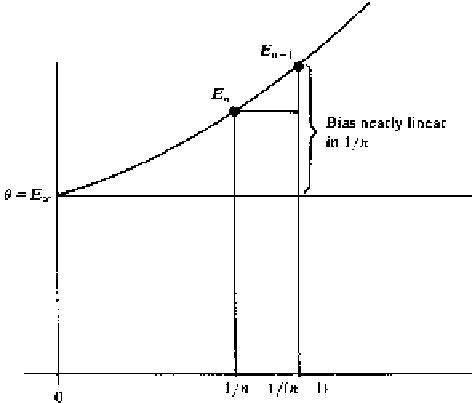
Search WWH ::

Custom Search