Geoscience Reference
In-Depth Information
Fast streamline simulations
on reconciled models
Fast streamline simulations
on reconciled models
Non-linear Euclidean
domain
Non-linear Euclidean
domain
Dissimilarity
matrix
Dissimilarity
matrix
Dissimilarity-distance
matrix
Dissimilarity-distance
matrix
1
1
1
E
E
13
13
13
12
12
12
MDS
MDS
3
3
3
2
2
2
23
23
23
Kernel PCA
Kernel PCA
Linear Feature
domain
Linear Feature
domain
E
E
Clustering and
pre-image
construction
Clustering and
pre-image
construction
F
F
Full-physics
simulation
Full-physics
simulation
RF
P10
RF
P10
RF
P10
RF
P50
RF
P50
RF
P50
RF
P90
RF
P90
RF
P90
= simulated model
(closest to the cluster centroids)
= simulated model
(closest to the cluster centroids)
Fig. 13. Workflow diagram using Multi Dimensional Scaling (MDS), k-PCA and k-means
clustering (modified from Scheidt and Caers, 2009 for three model realizations).
The workflow of Scheidt and Caers, 2009 incorporates a classical variant of metric MDS
where intervals and ratios between points are preserved in a manner of highest fidelity and
where it is assumed that the pattern-dissimilarity distances directly correspond to distances
in the
E
space. MDS finds the appropriate coordinates consistent with Euclidean distance
measure and the resulting map is governed exclusively by the pattern-dissimilarity
distances. Subject to the condition, that the distances are strongly correlated with the
dynamic response
r
, points within close proximity of each other exhibit similar recovery
characteristics, and hence, they are expected to contain similar geologic features. A
clustering algorithm is used to compartmentalize the Euclidean space into few distinct
clusters and to identify the realizations within the closest proximity of the cluster centroids.
The characteristic nonlinear structure of points in the
E
calls for kernel techniques such as
kernel Principal Component Analysis (k-PCA) (Schölkopf
et al.,
1996), where prior to
clustering nonlinear domains are mapped into a linear domain. When the MDS data are
mapped into a linear, high-dimensional feature domain
F
, using appropriate kernel method
e.g.
k-PCA, cluster analysis, such as
k-
means clustering (Tabachnick and Fidell, 2006) may
be applied to further compartmentalize the feature space F in order to identify cluster
centroids, where centroid of a cluster corresponds to a point whose parameter values are the
mean of the parameter values of all the points in the clusters. The pattern-dissimilarity
distances θ, closest to the cluster centroids in the Euclidean space are defined with respect to
recovery factor (RF) responses of two individual realizations
k
and
l
at times
t
T
, following




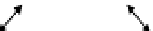



































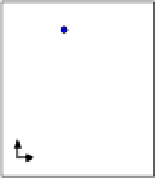




































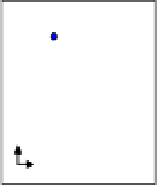





































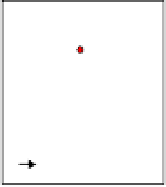









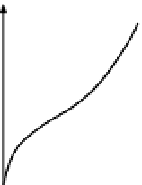








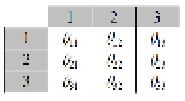









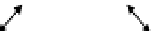



































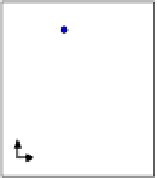




































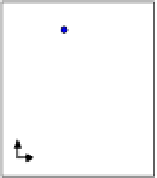





































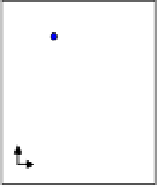




































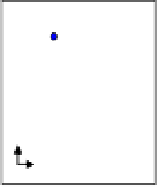






































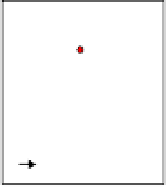






































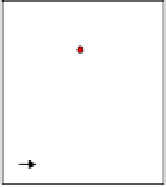











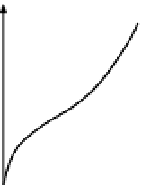















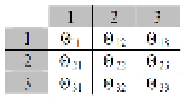
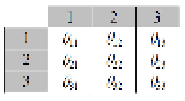


Search WWH ::

Custom Search