Geoscience Reference
In-Depth Information
- Either the concern is about conventional descriptive or inferential statistical
methods (regression model and data analysis), used on geographical data, to model,
for example, the relationships between the attributes of a set of spatial units. In some
cases, the results can be mapped, and the spatial organization is then analyzed a
posteriori by the domain expert. Regarding change, either it may be contained in the
analyzed variables (series of stamped variables or temporal variations), or analyses
are conducted separately for each time step. Here, the results are also evaluated and
compared
a posteriori
in order to apprehend change.
- Either the concern is about spatial statistical methods, developed to analyze
spatial distributions (for example, nearest neighbor analysis) or spatial
configurations (for example, spatial autocorrelation). In the latter case, an indicator
(or a hypothesis test) is estimated that characterizes the shape of the spatial
organization of the phenomenon that we are interested in at each date. Change can
then be interpreted through the indicator being observed during such a summary.
This categorization that is based on the role given to space is obviously very
schematic. Indeed, as we will see in Chapter 3, space may also be summarized in the
analyzed variables. Another categorization may be proposed in light of the
objectives of statistical processing: namely, either modeling in order to infer and
predict, or either modeling to describe, to filter or decompose the information.
Furthermore, in statistics, the analysis of time series, namely of observations
regularly spaced in time, has been the subject of developments of very emblematic
specific methods of the first type mentioned above. The models of time series enable
characterizing the distributions in time and making predictions. As an example, the
most known should be cited: the class of autoregressive models, based on the
regression model and that assumes that the past has an impact on what we are
observing in the present, and that the recent past is more influential than the distant
past. These models will allow, for example, expressing the Gross national product
(GNP) of a country in the current quarter, based on the different values of the
quarterly GNP over the last 5 years. Other explanatory variables can also be
introduced, measured according to their dynamics: in this example, the series of
values of the unemployment rate could be introduced to assess the explanatory
power of past values on the current value of the GNP. With these models, the
process is not modeled directly by the integration of the different states into the
model, but the tendency is modeled and a SPAN representation of the dynamics is
being approached. By analogy, a number of spatial models of the same type have
been developed, in line of the developments of Cliff
et al.
[CLI 73] on spatial
autocorrelation. They incorporate the fact that a value may be influenced by
neighboring values, close neighbors having more influence than the remote
neighbors. The pairing of these two classes of models has given rise to models
allowing the study of the explanatory power of factors evolving in time and space
[GRI 10].
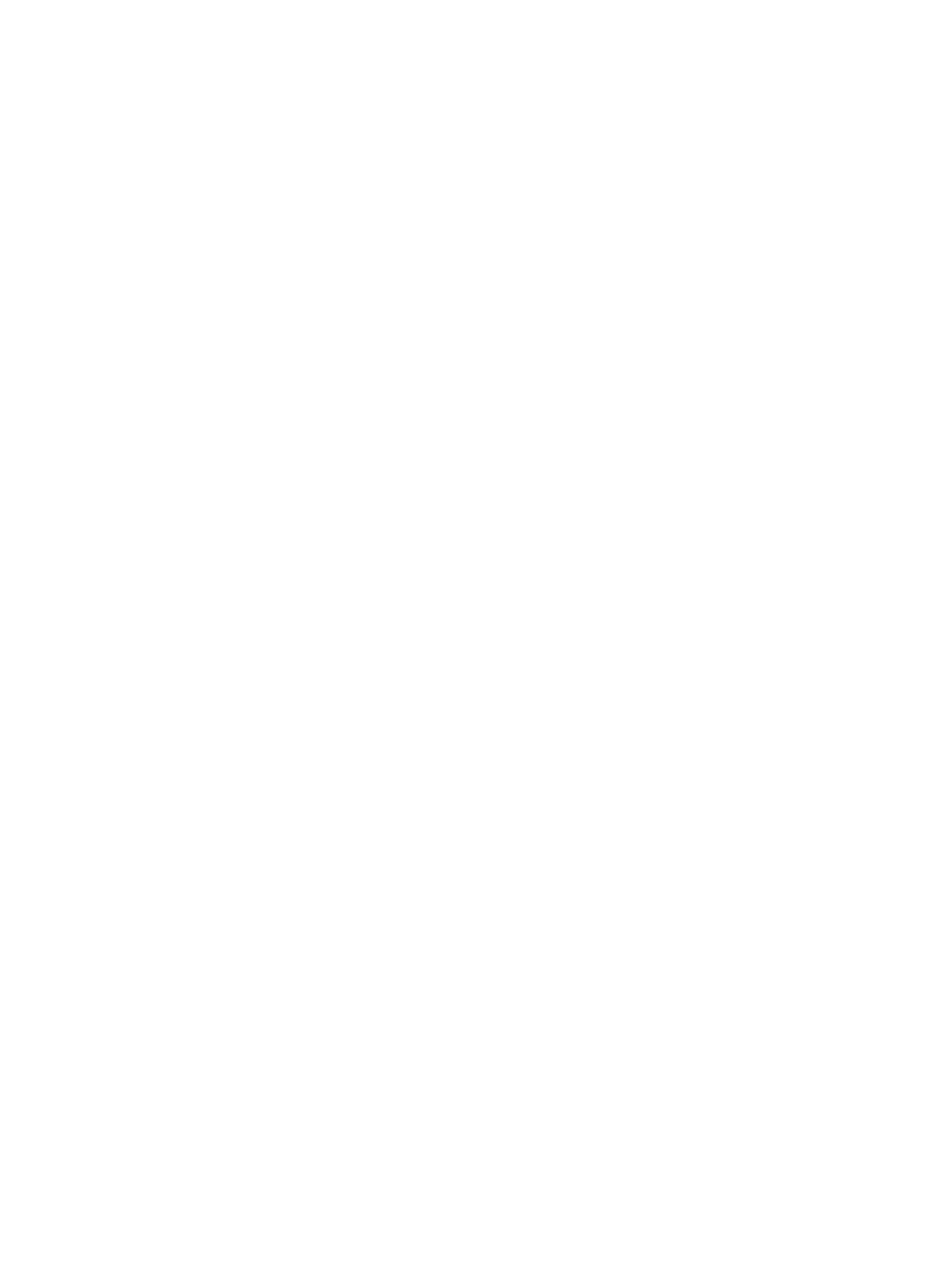
