Information Technology Reference
In-Depth Information
Fig. 4.
Dormitory-homophily of differ-
ent backbones, with sparsification ra-
tio 70%, (
y
-axis) compared to the ho-
mophily in the original network (
x
-axis)
for all Facebook100 networks. Points
above/below the dashed line indicate
homophily increase/decrease respective
the original network. Simmelian and
Quadrilateral homophily values for cor-
responding networks have been con-
nected by colored segments comparing
their performance.
better performance
Quadrilateral
Simmelian
Ⓩ
Ⓩ
Ⓩ
Ⓩ
Ⓩ
0.75
Ⓩ
Ⓩ
Random
Ⓩ
Ⓩ
Ⓩ
Ⓩ
Ⓩ
Ⓩ
Ⓩ
Ⓩ
Ⓩ
Ⓩ
Ⓩ
Ⓩ
Ⓩ
Ⓩ
Ⓩ
Ⓩ
Ⓩ
Ⓩ
Ⓩ
Ⓩ
Ⓩ
Ⓩ
Ⓩ
Ⓩ
Ⓩ
Ⓩ
Ⓩ
Ⓩ
Ⓩ
Ⓩ
Ⓩ
Ⓩ
Ⓩ
Ⓩ
Ⓩ
Ⓩ
Ⓩ
Ⓩ
Ⓩ
Ⓩ
Ⓩ
Ⓩ
Ⓩ
Ⓩ
Ⓩ
Ⓩ
Ⓩ
Ⓩ
Ⓩ
Ⓩ
Ⓩ
Ⓩ
Ⓩ
Ⓩ
Ⓩ
Ⓩ
Ⓩ
0.50
Ⓩ
Ⓩ
Ⓩ
Ⓩ
Ⓩ
Ⓩ
Ⓩ
Ⓩ
Ⓩ
Ⓩ
Ⓩ
Ⓩ
Ⓩ
Ⓩ
Ⓩ
Ⓩ
Ⓩ
Ⓩ
Ⓩ
Ⓩ
Ⓩ
Ⓩ
Ⓩ
Ⓩ
Ⓩ
Ⓩ
Ⓩ
Ⓩ
Ⓩ
Ⓩ
Ⓩ
Ⓩ
Ⓩ
Ⓩ
Ⓩ
Ⓩ
Ⓩ
Ⓩ
Ⓩ
Ⓩ
Ⓩ
Ⓩ
Ⓩ
Ⓩ
Ⓩ
Ⓩ
Ⓩ
Ⓩ
Ⓩ
Ⓩ
Ⓩ
Ⓩ
Ⓩ
Ⓩ
Ⓩ
Ⓩ
Ⓩ
Ⓩ
Ⓩ
Ⓩ
Ⓩ
Ⓩ
Ⓩ
Ⓩ
Ⓩ
Ⓩ
Ⓩ
Ⓩ
Ⓩ
Ⓩ
Ⓩ
Ⓩ
Ⓩ
Ⓩ
Ⓩ
Ⓩ
Ⓩ
Ⓩ
Ⓩ
Ⓩ
Ⓩ
Ⓩ
Ⓩ
Ⓩ
Ⓩ
Ⓩ
Ⓩ
Ⓩ
Ⓩ
Ⓩ
Ⓩ
Ⓩ
Ⓩ
Ⓩ
Ⓩ
Ⓩ
Ⓩ
Ⓩ
Ⓩ
Ⓩ
Ⓩ
Ⓩ
Ⓩ
0.25
Ⓩ
Ⓩ
Ⓩ
Ⓩ
Ⓩ
Ⓩ
Ⓩ
Ⓩ
Ⓩ
Ⓩ
Ⓩ
Ⓩ
Ⓩ
Ⓩ
Ⓩ
Ⓩ
Ⓩ
Ⓩ
Ⓩ
Ⓩ
Ⓩ
Ⓩ
Ⓩ
Ⓩ
Ⓩ
Ⓩ
Ⓩ
0.1
0.2
0.3
0.4
0.5
homophily (original network)
4.2 Edge Embeddedness Methods
We compare different methods which assign a weight
w
:
E
ₒ
R
≥
0
to each edge
e
=(
u,v
)
E
depicting its embeddedness. All these methods are then extended
using our UMST approach to guarantee the connectivity, such that a layout can
be computed from the resulting graph. We use the following approaches to assign
aweighttotheedges.
Random:
Assigns uniform random weights, as base line.
Jaccard:
Jaccard coecient,
|N
(
u
)
∩N
(
v
)
|
|N
(
u
)
∪N
(
v
)
|
∈
, as proposed by Satuliri et al. [20].
Simmelian:
Triadic Simmelian backbone, as proposed by Nick et al. [17].
Quadrilateral:
Quadrilateral Simmelian backbone, based on our embeddedness
method, which accumulates triadic effects at an edge with quadrangles (Sect. 3).
Density:
Metric by Auber et al. [1] accumulating densities of different subgroups
in the local neighborhood.
Ground Truth:
Knowledge of class membership in the synthetic network is
used to assign directly a low value to inter-cluster edges and a big value to
intra-cluster edges.
4.3 Quality Metrics
In contrast to the synthetic networks there is no ground truth available for the
Facebook networks. This makes it hard to evaluate outcomes of the different
methods. Nevertheless, it was found that for many of the Facebook networks,
the housing structure (dormitory attribute) is very relevant for the underlying
formation of social relations [17,26]. We, therefore, use the dormitory attribute
as a reference for evaluation.
Assume that we know the ground truth, meaning the class membership
c
(
v
)of
each vertex. A
perfect
algorithm, for example, would first remove all inter-cluster
edges before starting to remove intra-cluster edges while obeying the required
sparsification ratio. Since inter-cluster edges are removed priorly, this increases
the ratio between intra-cluster or homophily edges and the total number of edges.
If the edge embeddedness methods perform similar to this, the ratio of ho-
mophily edges
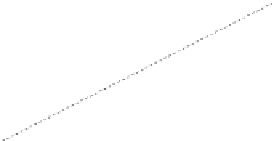

































































































































































































