Image Processing Reference
In-Depth Information
FIGURE 5
Performance comparison (accuracy and performance computation vs number of
trees of random forest).
6 Conclusion and Future Work
This chapter describes our method for automatic classification of protein crystals in crystalliza-
tion trial images. Our method focuses on extracting geometric features related to blobs, edges,
and lines from the images. Using random forest classifier, we are able to achieve classification
accuracy around 78% for a 4-class problem and this is a good classification performance.
This study focused on classifying a crystallization trial image according to the types of pro-
tein crystals present in the image. We only included images consisting crystals in our experi-
ments. In the future, we would like to perform a two-level classification. First, we plan to clas-
sify the images as non-crystals, likely-crystals, and crystals. Next, we plan to classify the sub-
categories. The current work will fall under the sub-classification of crystals.
We plan to improve the accuracy of the system further. The performance of our system de-
pends on the accuracy of image binarization. In some images, the thresholded images do not
capture the shapes of crystals correctly. Therefore, the features extracted from blobs may not
necessarily represent crystals. Because of this, the features extracted from those blobs are not
useful. To solve this problem, we plan to investigate different thresholding techniques. Our
initial study shows that using the best thresholded image for feature extraction improves the
classification performance.
References
[1] Pusey ML, Liu Z-J, Tempel W, Praissman J, Lin D, Wang B-C, et al. Life in the fast
lane for protein crystallization and X-ray crystallography.
Progr Biophys Molecul Biol.
2005;88(3):359-386.
[2] Cumbaa CA, Lauricella A, Fehrman N, Veatch C, Collins R, Luft J, et al. Automatic
classification of sub-microlitre protein-crystallization trials in 1536-well plates.
Acta
Crystallograp D Biol Crystallograp.
2003;59(9):1619-1627.
[3] Cumbaa C, Jurisica I. Automatic classiication and patern discovery in high-through-
put protein crystallization trials.
J Struct Funct Genom.
2005;6(2-3):195-202.
[4] Berry IM, Dym O, Esnouf R, Harlos K, Meged R, Perrakis A, et al. Spine high-
throughput crystallization, crystal imaging and recognition techniques: current state,
performance analysis, new technologies and future aspects.
Acta Crystallograph D Biol
Crystallograp.
2006;62(10):1137-1149.
[5] Pan S, Shavit G, Penas-Centeno M, Xu D-H, Shapiro L, Ladner R, et al. Automated
classification of protein crystallization images using support vector machines with
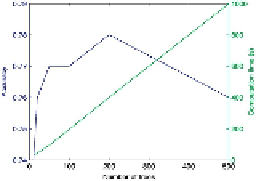
Search WWH ::

Custom Search