Information Technology Reference
In-Depth Information
Figure 10. WordComplete™ (top) and AdapTxt™
(centre and bottom)
based on more complex language models word
suggestion and completion can be beneficial with
novice users increasing typing speed by around
35% using AdapTxt™ suggestions on a soft key-
board (Dunlop, Glen, Motaparti, & Patel, 2006).
AdapTxt language models also self-tune over time
by learning patterns of use in the user's language
to further improve suggestions for the individual
user and his/her context of use (Figure 10 centre
and right shows off the shelf suggestions and those
after repeating a single phrase).
Gesture-Based Input
Gesture-based interaction attempts to combine the
best of visual keyboards with easy-to-remember
stylus movements to gain faster and smoother,
while still easy-to-learn, text entry. Building on
our motor-memory for paths, approaches such as
Cirrin (Mankoff & Abowd, 1998), Quikwriting
(Perlin, 1998) and Hex (Williamson & Murray-
Smith, 2005) are based on the user following a
path
through
the letters of the word being en-
tered (Figure 11). For on-screen approaches this
achieves faster entry rates than single character
printing with reduced stress and fatigue when
writing. Furthermore, in the case of Hex, the
approach can be used in one-handed on devices
with accelerometers/tilt sensors.
Gestures can be combined with more conven-
tional soft keyboards so that users can choose to
be dominated by extra time reading and reacting
to on-screen suggestions. We (Dunlop & Crossan,
2000) estimated that simple word completion
would reduce keystrokes by 17% but our model-
based evaluation (see section 4.1) predicted an
approximate halving of entry speed once user
interruption time was taken into account. Some
recent advances, however, have shown that when
Figure 11. Quikwriting (left) and the Hex entry for “was” (omitting letter display)(right)

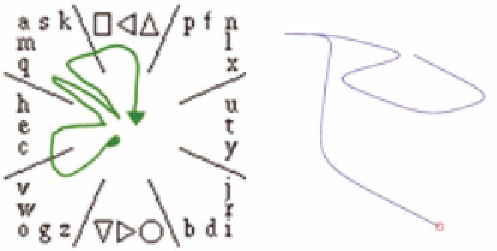
Search WWH ::

Custom Search