Graphics Reference
In-Depth Information
averaging or to concentrate on the selection of the predictor in regression problems;
our models usually evolve to more and more complex ones.
he key idea in Bayesian statistics - as opposed tosimply “statistical modeling” or
“estimation” - is to work with posterior uncertainty in inferences. At the theoretical
level, this means using random variables; at a more practical level, this implies the
use of simulations that represent draws from the joint posterior distribution. his is
seen mostclearly inhierarchical modeling. Figure
.
shows anexample of thevisu-
alization ofposterioruncertainty inahierarchical logistic regressionmodel(Gelman
et al.,
).
Figure
.
.
Displaying a fitted hierarchical logistic regression model, along with inferential
uncertainty. Eight states are shown from a voting preference model Prob
logit
−
(
y
i
=
)=
(
α
j
[
i
]
+
X
i
β
)
that includes all
US states. he solid black line is the median estimate for the probability that
a survey respondent in that state supported George Bush in his presidential campaign in
. he gray
lines represent random draws from the posterior distribution of the logistic regression coe
cients. he
dots shown are the observed data (zeros and ones), vertically jittered to make them more
distinguishable. his figure demonstrates several principles of Bayesian visualization: (
) small
multiples: parallel plots display the hierarchical structure of the data and model; (
) graphs are used to
understand the fitted model; (
) the fitted model and the data are shown on the same graph;
(
) posterior uncertainty is displayed graphically. A principle of Bayesian inference is also illustrated:
there were no data for Alaska but we were still able to draw predictions about the voting preference in
this state from the model. General principles of “good graphics” are used: a common scale is used for
all graphs, with bounds at
and
; axes are clearly labeled; jittering is employed (which works for
moderate sample sizes like that used in this example); thin lines and small dots are used. However here,
the small plots should be ordered by some meaningful criterion, such as by decreasing support for
Republicans, rather than alphabetically. he distribution of the linear predictor is skewed because the
most important single predictor by far was the indicator for African-Americans, which has an expected
value of
.
. he common scaling of the axes means that we do not actually need to label the axes on
each graph; however, we find that repeating the labels is convenient in this case. Labeling only some
graphs (as done for trellis plots) saves space but makes the graph more of a challenge to read, especially
when used as presentation graphics




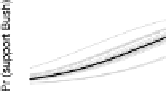


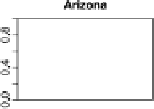

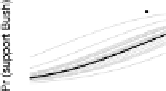























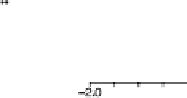

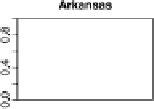

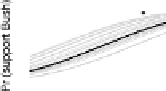












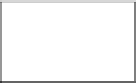



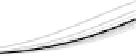






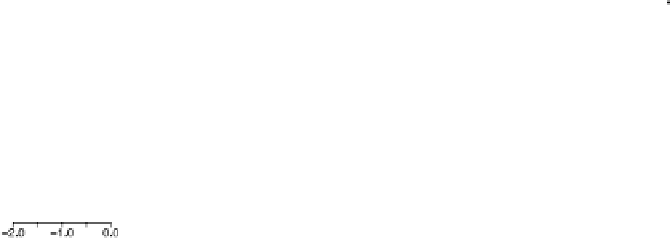
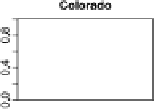

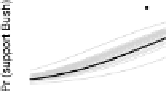


















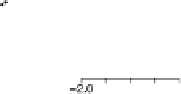



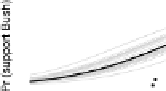




















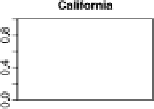

















































































































































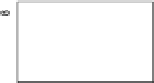



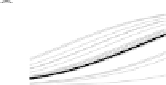

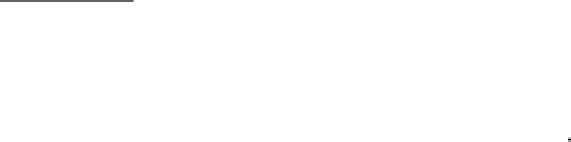






