Graphics Reference
In-Depth Information
in Fig.
.
, the ASH weights were sampled from the quadweight kernel, K
(
t
)=
t
+
.Specifically,
(
−
)
K
(
j
m
)
w
m
(
j
)=
m
ċ
j
=−
m,...,
,...,m .
(
.
)
m
i
=−
m
K
(
i
m
)
Eachvariablewasprebinnedinto
intervals.heASHsmoothingparameterswere
picked by eye to smooth out most of the noisy structure. All of these estimates are
multimodal. Regions of high elevation are somewhat unique climatically.
Next, the bivariate ASH density estimates wereconstructed in the remaining pan-
els of Fig.
.
. he data were prebinned into
intervals. A product version
of the ASH using the quadweight kernel was selected. Again, the structure is more
complexthan asingleGaussian andmorecomplexthanamixtureofafewGaussians.
Finally, all three variables were examined simultaneously in one ASH (Fig.
.
).
he data were prebinned into a mesh of size
and the quadweights again
employed. he shell displayed corresponds to α
%. he structure is quite fasci-
nating. Of course, given the huge dataset, one has high confidence that the features
clearly visible are not due to noise alone.
Without the availability of color in the figure,adding other contour levels is ill ad-
vised for such complex contours. Instead, a sequence of slices of the trivariate ASH
may be displayed (Fig.
.
). he climate is less complex near sea level and high al-
titudes. It would be interesting to link features in these frames with geographical in-
formation. Such research has been shown to be fruitful (Whittaker and Scott,
).
Without color, attempts to show
-D and
-D densities are best postponed. How-
ever, it should be clear from these examples the potential power that such displays
may bring. hese figures bring both an overview and yet great detail. Given the in-
creasing number of massive datasets available for analysis, these tools can prove
highly effective in the hands of a trained analyst.
=
Figure
.
.
Trivariate density estimate (ASH) of elevation (x), precipitation (y), and maximum
temperature (z)






































































































































































































































































































































































































































































































































































































































































































































































































































































































































































































































































































































































































































































































































































































































































































































































































































































































































































































































































































































































































































































































































































































































































































































































































































































































































































































































































































































































































































































































































































































































































































































































































































































































































































































































































































































































































































































































































































































































































































































































































































































































































































































































































































































































































































































































































































































































































































































































































































































































































































































































































































































































































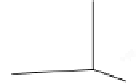


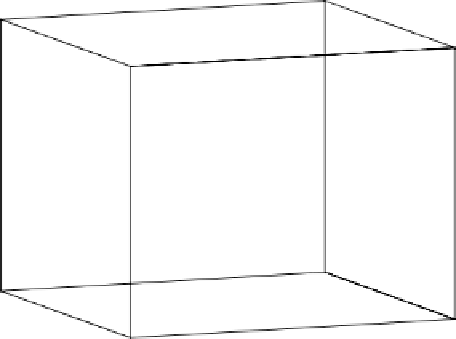

































































































































































































































































































